Modifications des communautés phytoplanctoniques et zooplanctoniques
Contexte
Le plancton (algues et animaux microscopiques) constitue la base des réseaux trophiques marins, il s’agit donc d’un indicateur important de l’état des écosystèmes. La brièveté des générations, la petite taille et, pour le phytoplancton, la dépendance directe à l'égard des nutriments dissous, font que le plancton est bien adapté à la détection des changements environnementaux. Les modifications des communautés planctoniques peuvent affecter des niveaux plus élevés des réseaux trophiques, tels que les mollusques et crustacés, le poisson et les oiseaux de mer, puisque ces organismes dépendent directement ou indirectement du plancton. On peut utiliser des indicateurs se fondant sur des formes de vie du plancton - des organismes possédant les mêmes traits fonctionnels (Figure 1) - pour révéler les réactions des communautés planctoniques à des facteurs tels que la charge de nutriments d’origine anthropique et les modifications entraînées par le climat. Les modifications de l'abondance des formes de vie du plancton sur de courtes périodes peuvent avoir des conséquences immédiates pour les niveaux trophiques supérieurs, tandis que les changements progressifs sur plusieurs décennies peuvent indiquer des modifications à long terme d'aspects importants du fonctionnement de l'écosystème.
À l’échelle régionale de l’Atlantique du Nord-Est, les modifications des communautés planctoniques sont fortement liées aux conditions environnementales. Les habitats pélagiques peuvent être définis en fonction de caractéristiques clés de la colonne d'eau, telles que la profondeur, l'influence de l'eau douce, la stratification, et sur la base de schémas spatialement cohérents de la dynamique du phytoplancton. L'examen de la direction et de l'ampleur des changements dans l'abondance des formes de vie au fil du temps dans les habitats pélagiques peut révéler des schémas spatiaux de changement environnemental. De même, les tendances déterminées à partir de la surveillance régulière de stations fixes peuvent être comparées aux tendances observées dans les habitats pélagiques environnants et adjacents. Cette évaluation porte sur les liens plausibles entre les pressions environnementales et les changements à long terme dans les communautés de plancton.
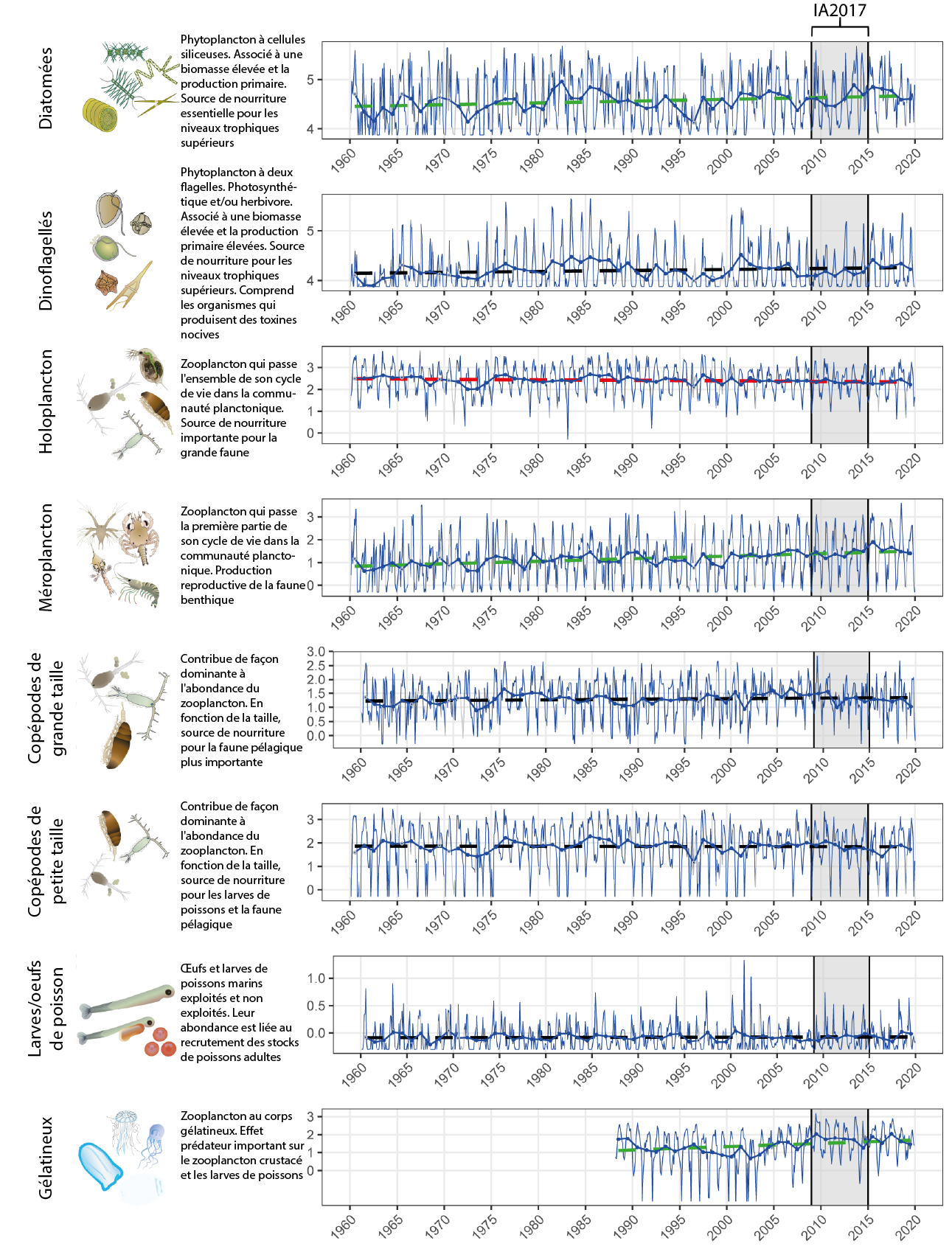
Figure 1 : Séries temporelles mensuelles et annuelles à long terme de l'abondance transformée en log10 pour huit formes de vie du plancton dans la Manche occidentale. Les lignes bleues indiquent la variabilité mensuelle (ligne plus fine) et l'abondance moyenne annuelle (ligne plus épaisse). Les lignes en pointillé indiquent les tendances linéaires de l'abondance annuelle sans aucune déduction sur la signification statistique. Le test de tendance de Kendall est utilisé pour déduire la signification des tendances, le rouge représentant une tendance à la baisse, le vert une tendance à la hausse et le noir une absence de tendance. Données provenant de l'étude Continuous Plankton Recorder (CPR) et du Plymouth Marine Laboratory (pour le zooplancton gélatineux uniquement en raison de la capture non quantitative des taxons gélatineux par le CPR). La région ombrée représente la période de l'étude IA2017. Les images de plancton sont une gracieuseté du réseau d'intégration et d'application du Centre des sciences de l'environnement de l'Université du Maryland.
Indicators based on plankton lifeforms have been used to assess community response to sewage pollution (Charvet et al 1998; Tett et al., 2008), anoxia (Rakocinski 2012), fishing (Bremner et al., 2004), eutrophication (HELCOM 2012), climate change (Beaugrand 2005; Bedford et al., 2020a; McQuatters-Gollop et al., 2019), and ocean acidification (Keys et al., 2018). Indicators based on functional groups have been proven relevant for the description of the community’s structure and biodiversity and are more easily inter-compared than other indicators based on taxonomy (Estrada et al., 2004; Gallego et al., 2012; Garmendia et al., 2012; Mouillot et al., 2006).
In practice, it is often preferable to aggregate species with similar traits into functional groups, such as lifeforms (Figure 1), rather than assessing the dynamics of individual species. Measures of species abundance are frequently subject to large interannual and regional variation, often due to natural physical dynamics and habitat preferences rather than anthropogenic stressors (de Jonge, 2007). Functional group abundance is often less variable because variability in the abundances of the group’s constituent species averages out. Cryptic speciation (species with near-identical appearance) within the plankton community, alongside the limitations of identifying plankton using routine light microscopy techniques, make it difficult to generate accurate counts at a species or genus level. Functional group abundance is more reliable as many plankton lifeforms are easily identified, making comparisons between different laboratories and institutes feasible. Both abundance and biomass data can be used to inform lifeform time series, depending on the lifeform in question and data availability from monitoring programmes.
In addition to studying change in individual lifeforms, these concepts can be extended to investigating changes in ecologically relevant pairs of lifeforms in the form of a lifeform pairs index. The precise combination of lifeforms composing the pairs will depend on the habitat and the objective of the indicator, e.g., as a measure for change in pelagic habitats, food webs, seafloor integrity or eutrophication. Change in the abundance of ecologically linked lifeforms over time can also provide an indication of changes in various aspects of ecosystem function. These include, for example: the transfer of energy from primary to secondary producers (changes in phytoplankton and zooplankton); the pathway of energy flow and top predators (changes in gelatinous zooplankton and fish larvae); benthic / pelagic coupling, i.e., changes in holoplankton (fully planktonic) and meroplankton (only part of the lifecycle is planktonic, the remainder is benthic) (see also Gowen et al., 2011; McQuatters-Gollop et al., 2019).
While studying lifeform pairs can be helpful for detecting changes in the annual cycle of ecologically linked lifeforms, assessing lifeforms separately is more suitable for evaluating gradual change over time. It is simpler to interpret results of an analysis of correlation between lifeform abundance and environmental pressures, than between an index of lifeform pairs and environmental pressures (Bedford et al., 2020a). A method has been developed to detect potential links between environmental pressures and change in lifeform abundance over time. The main features of the method are: (i) the grouping of planktonic taxa into functional types or lifeforms; (ii) spatially division of plankton samples to construct distinct time-series of lifeform abundance; (iii) using a robust nonparametric test to quantify long term changes in lifeform abundance; (iv) relating change in lifeform abundance to trends in environmental pressures and climate indices.
The OSPAR Intermediate Assessment 2017 (IA2017) stated that conclusions on the trends observed could not yet be made as additional work was needed to investigate environmental pressures and potential impacts of climate change and other human pressures on plankton communities. This QSR 2023 assessment addresses plausible links between environmental pressures and long term changes in plankton communities.
Previous assessment
The previous assessment (Intermediate Assessment 2017; IA2017) was based largely on state space theory (see below) and examined differences in the relative abundances of ecologically relevant lifeform pairs between a past comparison period (2004 to 2008) and a contemporary assessment period (2009 to 2014). While this method is suitable for detecting changes in the annual cycle of ecologically linked lifeforms, it is less suited to evaluating gradual change over time. Further, stronger statistical links can be drawn by examining relationships between the abundances of distinct planktonic lifeforms and environmental pressures, than between an index of lifeform pairs and environmental pressures (Bedford et al., 2020a). For the current assessment a simplified approach was developed, based on measuring long-term changes in the abundance of key lifeforms and evaluating how environmental pressures co-varied with changes in lifeform abundance. A long-term perspective helped improve confidence in establishing links to environmental pressures. Short-term changes within the assessment period were also evaluated to identify emerging trends. The PH1 indicator as originally defined in IA2017 was still evaluated for this assessment and is described under the Lifeform pairs indicator approach section of the Assessment Methods and the respective results are described in the Results (extended) section.
Kendall trend test and statistic
Abundance trends for planktonic lifeforms can be evaluated over time by applying the Kendall trend test to annual mean abundance values (Bedford et al., 2020a; Desmit et al., 2020). The test was performed on annual log10 transformed mean abundance values, rather than monthly or seasonal values, to remove the seasonal variation typical of plankton time series data (Figure 1). This nonparametric test generates a statistic which is derived by comparing each value in a time-series with each of the values preceding it. If a latter value is greater than a previous one, the pairwise comparison is assigned a value of 1. If it is lower it is assigned a value of -1, with 0 assigned to cases when there is no difference between values. The sum of the pairwise comparisons for the time-series produces Kendall’s S-statistic. The variance in the S-statistic is used to derive a Z-score with an approximately normal distribution; thus, confidence in this statistic can be assessed with an associated p-value, with p ≤ 0,05 generally accepted as statistically significant change. The sign of the test statistic reveals the direction of the trend, with a positive statistic indicating an increasing trend and a negative statistic indicating a decreasing trend. The magnitude of the statistic is proportional to the strength of the trend. A great benefit of this nonparametric test is that it yields identical results irrespective of the data transformation method and is not sensitive either to gaps in data or to non-linear or irregular trends. It also has the advantage that abundance trends are comparable across datasets, lifeforms, and assessment units.
Time-series plots (Figure 1) indicate variation in lifeform abundances through time for a single assessment unit, with the blue lines displaying monthly variability (thinner line), and annual mean abundance (thicker line) values used to derive the Kendall statistic. Data correspond to the assessment unit representing the western entrance to the English Channel (‘Channel well mixed’ see Figure a) and contains the fixed-point station ‘L4’, consistently monitored by the Plymouth Marine Laboratory since 1988.
The ability to aggregate complex plankton data into ecologically relevant lifeforms is a useful tool for assessment because it can help identify where changes are occurring (McQuatters-Gollop et al., 2019). The ability to understand which taxa are driving changes in a lifeform can also be valuable. By calculating the Kendall statistic for each taxon, additional information can be gleaned to assist in interpreting lifeform time-series trends. For example, Figure a displays change in the abundance of diatoms for the ‘Channel well mixed’ COMP4 assessment unit described in Figure 1. The abundance of diatoms has been increasing since 1960 (z = 2,66, p ≤ 0,05). Through examining the annual abundance time-series for individual diatom taxa it becomes possible to also conclude that overall trend of increasing diatom abundance is driven by positive abundance trends in 11 out of 54 diatom taxa. Increasing trends and greater absolute abundances for Proboscia alata and Thalassiosira spp indicate that they are predominantly responsible for the increasing abundance trend in diatoms. Additionally, while the net trend is increasing, four diatom taxa (Guinardia striata, Skeletonema costatum, Thalassionema nitzschioides, and Odontella sinensis) are actually decreasing in abundance.
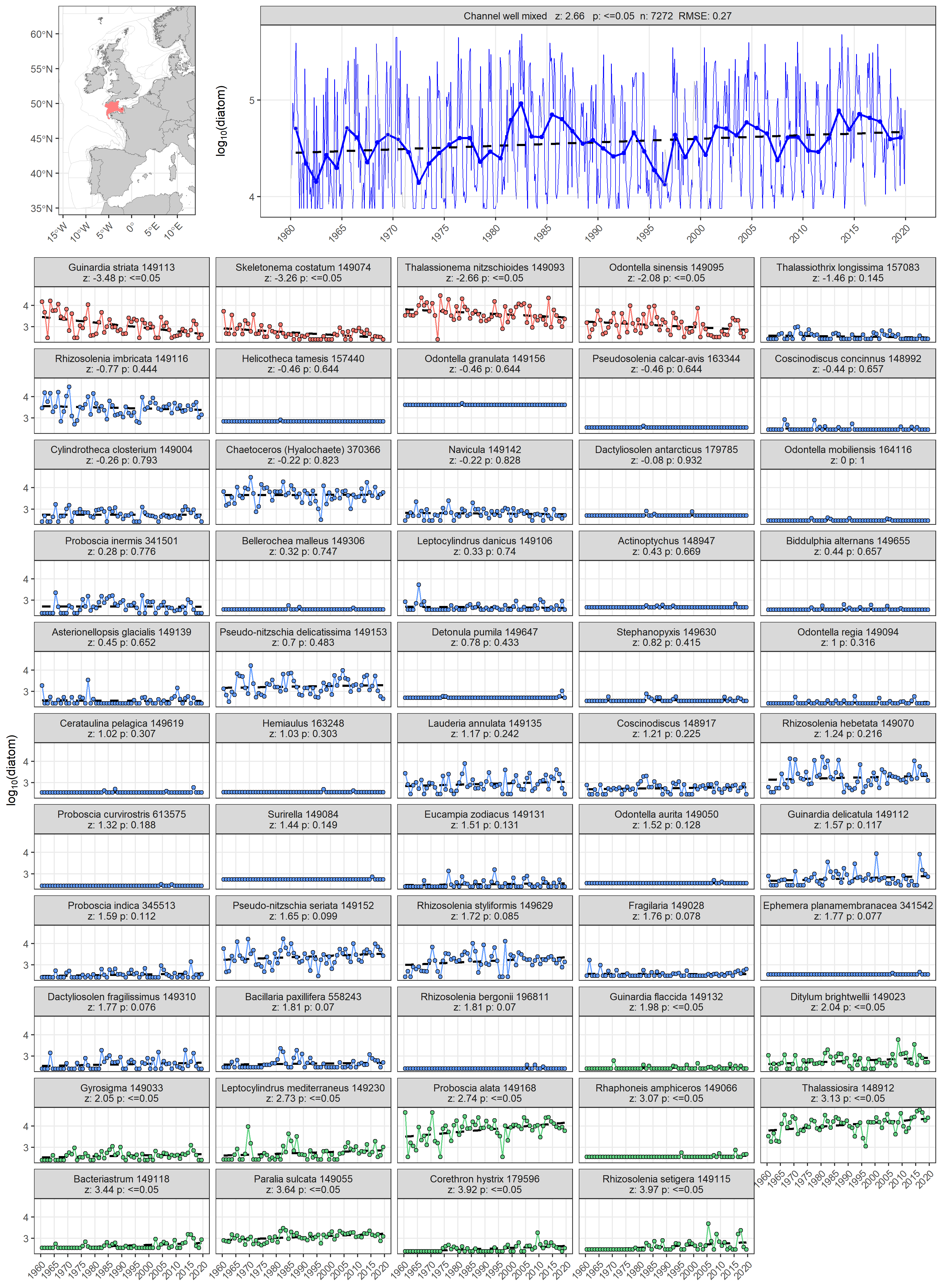
Figure a: Long-term log10 transformed abundance time-series for diatoms in the ‘Channel well mixed’ COMP4 assessment unit. Data obtained from the Continuous Plankton Recorder (CPR) survey. Individual diatom taxa abundance time series are displayed as facets. Facet labels indicate taxon scientific name and Aphia ID, z: derived from Kendall’s S-statistic, and p: Kendall p-value. Taxon time-series are coloured according to the outcome of the Kendall trend test, with red: decreasing trend and p ≤ 0,05, blue: p > 0,05 (no trend), green: increasing trend and p ≤ 0,05.
Since this assessment was based on data from multiple sources, the Kendall statistic was calculated independently for each combination of dataset, assessment unit, and lifeform. Since the datasets used also varied in duration, to avoid discarding data the full duration of each dataset was assessed up until the end of 2019. For a dataset to be included in this analysis, it needed to contain samples collected within the assessment period (2015-2019) and prior to the assessment period.
Spatial Scale:
Because plankton community composition, distribution, and dynamics are closely linked to their environment, the analysis was performed at the scale of the ‘COMP4 assessment units’ (COMP4 v8a; Figure b, Table a). Assessment units within the Greater North Sea and Celtic Seas (OSPAR Regions II and III, respectively) were initially developed by Deltares and partner institutes as part of the EU ‘Joint Monitoring Programme of the Eutrophication of the North Sea with Satellite data’ (JMP-EUNOSAT; Enserink et al., 2019) and further refined in the revision process of the eutrophication assessment by OSPAR expert groups ICG-EMO and TG-COMP. Assessment units with similar phytoplankton dynamics were derived from cluster analysis of satellite data for chlorophyll a and primary production. Boundaries between assessment units were derived by relating clustering results to the best-matching gradients in environmental variables obtained from the three-dimensional hydrodynamic Dutch Continental Shelf model version 6 (DCSMv6 FM). The variables which best matched the divisions highlighted by clustering were depth, salinity, and stratification regime. Additional geographic areas were added such as the Channel, Irish Sea, and Kattegat. These assessment units are a geographical representation of the conditions most likely to drive plankton distribution, dynamics, and community composition. The assessment units are being regularly updated and may not perfectly reflect the true state of the pelagic environment in their present form.
Figure b: COMP4 assessment units developed by JMP-EUNOSAT (Enserink et al., 2019) and OSPAR. Area codes displayed in this figure are referred to in Table a. https://odims.ospar.org/en/submissions/ospar_comp_au_2023_01/
COMP 4 assessment units categories | Area code | Area name | Mean salinity at surface (PSU) | Mean depth (m) | OSPAR Region |
---|---|---|---|---|---|
Variable salinity assessment units | ADPM | Adour plume | 34,4 | 87 | IV |
ELPM | Elbe plume | 30,8 | 18 | II | |
EMPM | Ems plume | 31,4 | 19 | II | |
GDPM | Gironde plume | 33,5 | 34 | IV | |
HPM | Humber plume | 33,5 | 16 | II | |
LBPM | Liverpool Bay plume | 30,6 | 15 | III | |
LPM | Loire plume | 33,8 | 38 | IV | |
MPM | Meuse plume | 29,3 | 16 | II | |
RHPM | Rhine plume | 31,0 | 17 | II | |
SCHPM1 | Scheldt plume 1 | 31,4 | 13 | II | |
SCHPM2 | Scheldt plume 2 | 30,9 | 15 | II | |
SHPM | Shannon plume | 34,1 | 61 | III | |
SPM | Seine plume | 31,8 | 25 | II | |
THPM | Thames plume | 34,4 | 22 | II | |
Coastal assessment units | CFR | Coastal FR channel | 34,2 | 33 | II |
CIRL | Coastal IRL 3 | 34,0 | 65 | III | |
CNOR1 | Coastal NOR 1 | 34,3 | 190 | II | |
CNOR2 | Coastal NOR 2 | 34,0 | 217 | II | |
CNOR3 | Coastal NOR 3 | 32,4 | 171 | II | |
CUK1 | Coastal UK 1 | 34,5 | 60 | III | |
CUKC | Coastal UK channel | 34,8 | 37 | II | |
CWAC | Coastal Waters AC | No information | No information | IV | |
CWBC | Coastal Waters BC | No information | No information | IV | |
CWCC | Coastal Waters CC | No information | No information | IV | |
ECPM1 | East Coast (permanently mixed) 1 | 34,8 | 73 | II | |
ECPM2 | East Coast (permanently mixed) 2 | 34,5 | 43 | II | |
GBC | German Bight central | 33,4 | 39 | II | |
IRS | Irish Sea | 33,7 | 65 | III | |
KC | Kattegat Coastal | 25,7 | 21 | II | |
KD | Kattegat Deep | 27,6 | 50 | II | |
NAAC1A | Noratlantic Area NOR-NorC1 | No information | No information | IV | |
NAAC1B | Noratlantic Area NOR-NorC1 | No information | No information | IV | |
NAAC1C | Noratlantic Area NOR-NorC1 | No information | No information | IV | |
NAAC1D | Noratlantic Area NOR-NorC1 | No information | No information | IV | |
NAAC2 | Noratlantic Area NOR-NorC2 | No information | No information | IV | |
NAAC3 | Noratlantic Area NOR-NorC3 | No information | No information | IV | |
OC | Outer Coastal DEDK | 33,4 | 27 | II | |
SAAC1 | Sudatlantic Area SUD-C1 | No information | No information | IV | |
SAAC2 | Sudatlantic Area SUD-C2 | No information | No information | IV | |
SAAP2 | Sudatlantic Area SUD-P2 | No information | No information | IV | |
SNS | Southern North Sea | 34,3 | 32 | II | |
Shelf assessment units | ASS | Atlantic Seasonally Stratified | 35,2 | 134 | III, IV |
CCTI | Channel coastal shelf tidal influenced | 34,8 | 40 | II | |
CWM | Channel well mixed | 35,1 | 77 | II, III | |
CWMTI | Channel well mixed tidal influenced | 35,0 | 59 | II | |
DB | Dogger Bank | 35.,1 | 28 | II | |
ENS | Eastern North Sea | 34,8 | 43 | II | |
GBCW | Gulf of Biscay coastal waters | 34,6 | 53 | IV | |
GBSW | Gulf of Biscay shelf waters | 34,9 | 107 | IV | |
IS1 | Intermittently Stratified 1 | 35,3 | 138 | II, III | |
IS2 | Intermittently Stratified 2 | 35,1 | 102 | II | |
NAAP2 | Noratlantic Area NOR-NorP2 | No information | No information | IV | |
NAAPF | Noratlantic Area NOR-Plataforma | No information | No information | IV | |
NNS | Northern North Sea | 35,0 | 121 | II | |
NT | Norwegian Trench | 34,1 | 349 | II | |
SAAP1 | Sudatlantic Area SUD-P1 | No information | No information | IV | |
SK | Skagerrak | 31,8 | 134 | II | |
SS | Scottish Sea | 35,1 | 89 | II, III | |
Oceanic / beyond shelf assessment units | ATL | Atlantic | 35,3 | 2291 | II, IV, V |
NAAO1 | Noratlantic Area NOR-NorO1 | No information | No information | IV | |
OWAO | Ocean Waters AO | No information | No information | IV | |
OWBO | Ocean Waters BO | No information | No information | IV | |
OWCO | Ocean Waters CO | No information | No information | IV | |
SAAOC | Sudatlantic Area SUD-OCEAN | No information | No information | IV |
Fixed-point station categories | Area code | Data provider | Salinity (surface mean) | Depth (mean) | OSPAR Region |
---|---|---|---|---|---|
Variable salinity fixed-point stations | Norderney | NLWKN | No information | No information | II |
LPO | Scottish Association of Marine Science | No information | No information | III | |
Loch Ewe | Marine Scotland Science | No information | No information | III | |
Coastal fixed-point stations | L4 | Plymouth Marine Laboratory | No information | 54 | II |
NOVANA | Aarhus University | No information | No information | II | |
Scalloway | Marine Scotland Science | No information | No information | II | |
Scapa | Marine Scotland Science | No information | No information | II | |
Stonehaven | Marine Scotland Science | No information | No information | II | |
RADIALES | Instituto Espanol de Oceanografia | No information | No information | IV |
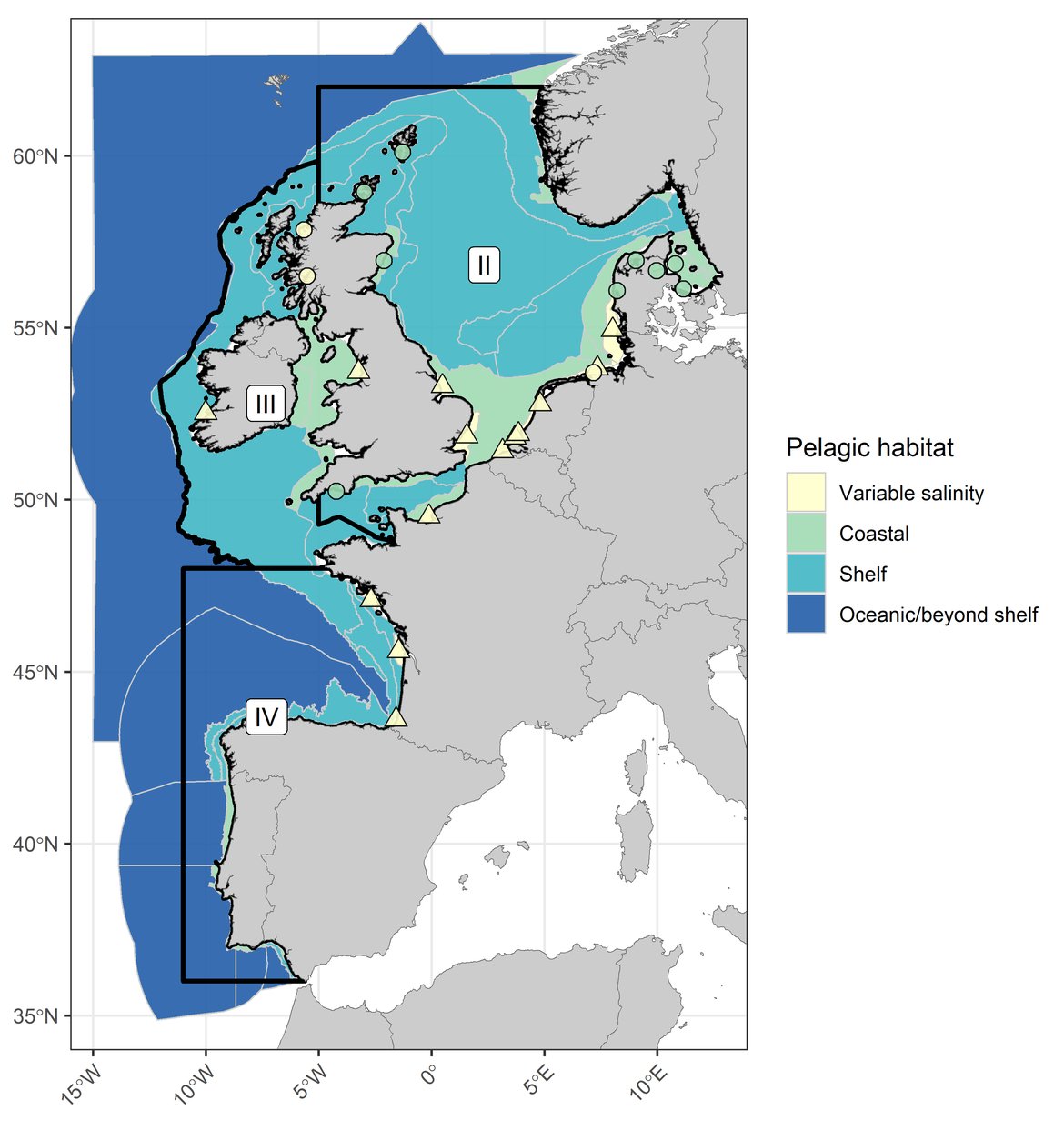
Figure c: The categorisation of COMP4 assessment units and fixed-point stations according to pelagic habitat type. Three assessed OSPAR Regions (I, II, and III) indicated by black outlines. River plumes are indicated by triangle symbols and fixed-point stations are indicated by circles.
Because the Bay of Biscay and Iberian Coast (OSPAR Region IV) extended beyond the boundaries of the DCSMv6 FM, assessment units within this Region were developed using a different methodology, based on phytoplankton dynamics (Spain) and salinity dynamics (Portugal). To delineate assessment units for the Spanish coast, a polygon was created to extend from the coast to the exclusive economic zone (EEZ) boundary. Daily NASA Aqua/MODIS Level-2 satellite images (https://doi.org/10.5067/AQUA/MODIS/L2/OC/2018) were used to calculate climatological mean values of chlorophyll a for each pixel. K-means clustering was then used to group pixels with similar dynamics, resulting in six distinct groupings within the main Spanish polygon. Portugal’s three Water Framework Directive assessment units were extended to the boundaries of the Portuguese EEZ. These assessment units were further divided longitudinally to separate pelagic waters from coastal waters more subject to eutrophication from river influence by applying a salinity threshold of 30,0–34,5 for coastal waters and >34,5 for offshore waters, followed by a bathymetry threshold of 100 m.
COMP4 assessment units and fixed-point stations were categorised according to the OSPAR Regions they intersected as well as the habitat type they represented (Table a, Table b, Figure c). The four habitat types considered were: variable salinity (representing areas of freshwater influence where estuarine plumes extend beyond waters designated as Transitional Waters under Directive 2000/60/EC), coastal (representing areas with mean salinity < 34,5 psu), shelf (representing areas with mean salinity > 34,5 and mean depth < 200 m) and oceanic / beyond shelf (representing areas with mean salinity > 34,5 and mean depth > 200 m).
Plankton Data:
The assessment has been carried out using 24 phytoplankton and zooplankton datasets from 14 sources (Table c, Figure d). Other datasets were provided but they were out of the scope of this assessment (e.g., time-series with comparison period duration shorter than assessment period duration, time-series ending prior to the assessment period, time-series commencing during assessment period, distributed sampling locations restricted to transitional waters only). These additional datasets were submitted by: Vlaams Instituut voor de Zee (BE), Bundesamt für Seeschifffahrt und Hydrographie (DE), Centre for Environment, Fisheries and Aquaculture Science (UK), Newcastle University (UK), and Natural Resources Wales (UK).
Contracting Party | Institute | Dataset name | Date range |
---|---|---|---|
Belgium (BE) | Vlaams Instituut voor de Zee (VLIZ) | LW_VLIZ_zoo | 2014-2020 |
Denmark (DK) | Aarhus University (AU) | NOVANA phytoplankton | 1985-2020 |
Germany (DE) | Landesamt für Landwirtschaft, Umwelt und ländliche Räume des Landes Schleswig-Holstein (LLUR) | OSPAR_LLUR-SH_2010-2020 | 2010-2020 |
Niedersächsischer Landesbetrieb für Wasserwirtschaft, Küsten und Naturschutz (NLWKN) | OSPAR_NLWKN_1999-19_phyto | 1999-2019 | |
Netherlands (NL) | Rijkswaterstaat (RWS) | RWS_Fpzout_2000-2019_phyto | 2000-2019 |
Portugal (PT) | Instituto Portuguêes do Mar e da Atmosfera (IPMA) | Pseudo-nitzschia vs Dinophysis_IPMA | 2002-2020 |
Spain (ES) | Instituto Español de Oceanografía (IEO) | IEO_RADIALES_Phyto | 1989-2016 |
IEO_RADIALES_Zoo | 1991-2018 | ||
Sweden (SE) | Swedish Meteorological and Hydrological Institute (SMHI) | National data_SMHI_Kattegat-Dnr: S/Gbg-2021_116_phyto | 1989-2021 |
National data_SMHI_Kattegat-Dnr: S/Gbg-2021_116_zoo | 1996-2020 | ||
National data_SMHI_Skagerrak-Dnr: S/Gbg-2021_116_phyto | 1986-2020 | ||
National data_SMHI_Skagerrak-Dnr: S/Gbg-2021_116_zoo | 1996-2020 | ||
United Kingdom (UK) | Centre for Environment, Fisheries and Aquaculture Science (Cefas) | Cefas SmartBuoy Marine Observational Network - UK Waters Phytoplankton Data 2001-2019 | 2001-2019 |
Environment Agency (EA) | EA PHYTO 2000-2020 | 2000-2020 | |
Marine Biological Association (MBA) | CPR dataset 1960-2019 | 1960-2019 | |
Marine Scotland Science (MSS) | MSS Scalloway Phytoplankton dataset | 2000-2018 | |
MSS Loch Ewe Phytoplankton | 2000-2020 | ||
MSS Loch Ewe zooplankton | 2002-2017 | ||
MSS Scapa Phytoplankton dataset | 2000-2020 | ||
MSS Stonehaven Phytoplankton | 2000-2020 | ||
MSS Stonehaven zooplankton | 1999-2020 | ||
Plymouth Marine Laboratory (PML) | PML_L4 phytoplankton | 1992-2020 | |
PML_L4 zooplankton | 1988-2020 | ||
Scottish Association for Marine Science (SAMS) | SAMS-LPO-Phyto-Dec2021 | 1970-1981, 2000-2017 |
The data submitted by AU (DK), PML (UK), MSS (UK), NLWKN (DE) and IEO (ES) were from discrete fixed-point stations which were evaluated independently. The data submitted by SAMS (UK) were from multiple stations within a small area of freshwater influence. These data were aggregated and treated as a single fixed-point time series.
Data from the Continuous Plankton Recorder (CPR) survey, collected by the Marine Biological Association (MBA, UK), consisted of spatially distributed data collected along transects. The CPR survey, provides offshore open ocean data at a broad spatial scale using ships-of-opportunity (Reid et al., 2003). Due to the distributed nature of CPR data, CPR samples are typically aggregated across a grid or a set of polygons at monthly temporal resolution (Bedford et al., 2020a). The remaining fixed-point datasets, including VLIZ (BE), LLUR (DE), RWS (NL), IPMA (PT), SMHI (SE), Cefas (UK), and EA (UK), were also treated in this manner since samples were collected at several distributed stations within close proximity to one another. The dataset from IPMA (PT) only records abundances for the genera Pseudo-nitzschia (diatom) and Dinophysis (dinoflagellate), thus results from the Portuguese data should only be considered as a proxy for diatom and dinoflagellate lifeform abundances.
Data from the different providers were not combined for analysis due to differences in sampling, plankton analysis and enumeration methods. Instead, the datasets were analysed separately. Each dataset has internal QA/QC procedures to ensure consistency and accuracy of the data. Before total lifeform abundance values were log10 transformed, a nominal value equivalent to half the minimum non-zero observed value for each time-series was added to each sample. All spatially distributed data (e.g., excluding AU, PML, MSS, IEO, NLWKN and SAMS) were averaged per month within each assessment unit. In a few cases, this resulted in localised groupings of samples being extrapolated across much larger assessment units (see distribution of CPR samples in Figure d (i)), as was the case for CPR data along the west of Scotland (Intermittently Stratified 1) and Ireland (Atlantic Seasonally Stratified). For months when there were no samples within an assessment unit, gaps in the time series were filled by extracting a mean value from an inverse distance weighted interpolated surface generated from samples adjacent to the assessment unit (<250 nmi) from the same dataset. Years containing less than eight months of averaged and spatially interpolated values were discarded. Remaining gaps of three months or less were filled via linear interpolation. For the reporting of trends and linking to pressures, in cases where multiple suitable datasets contained samples from within the same assessment unit the trends displayed were based on the dataset with the greatest number of unique months (e.g., June 1978, February 2000, May 2014).
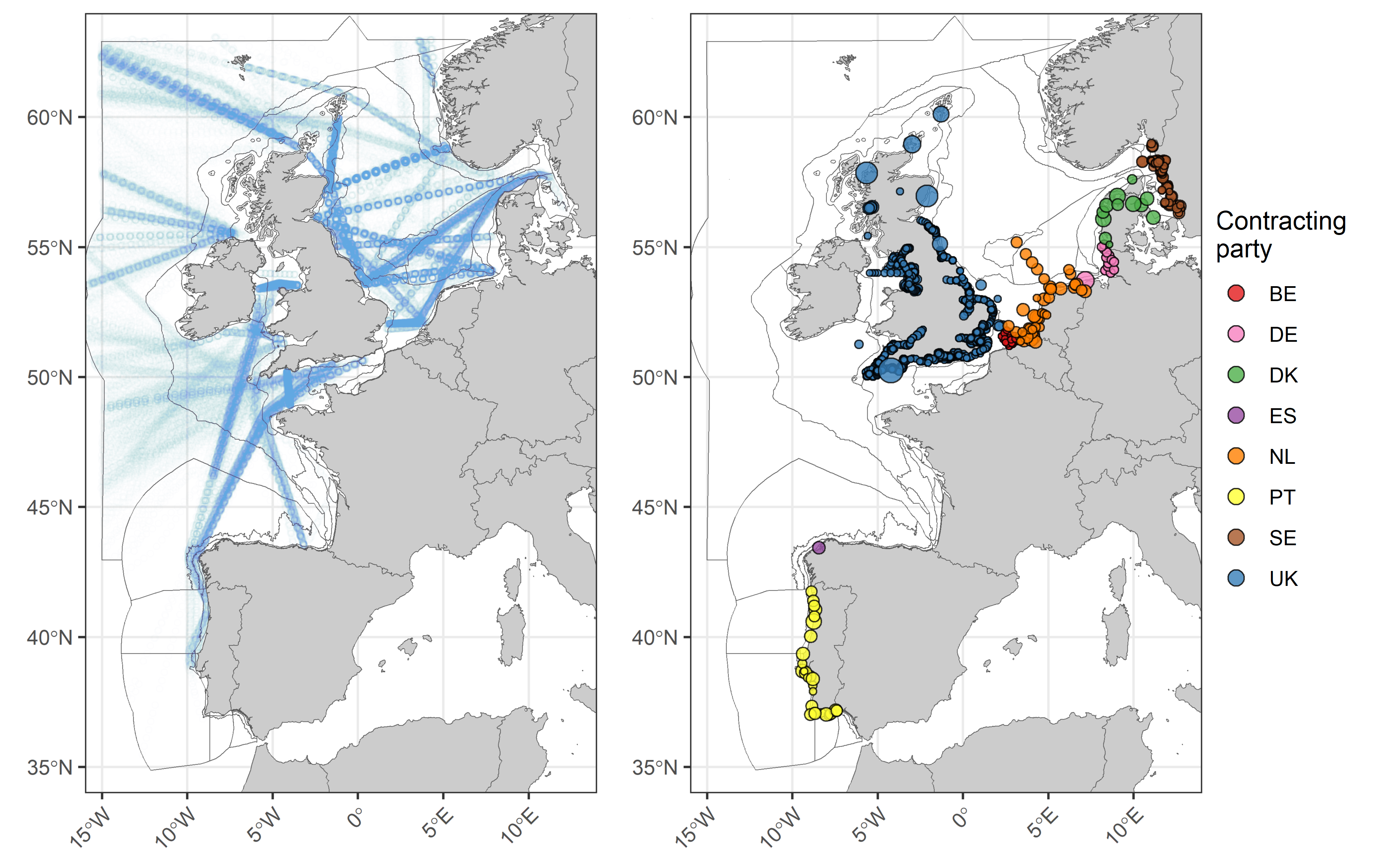
Figure d: CPR tracks and the locations of samples from all other datasets used in the assessment. For the locations, point size is proportional to the number of samples taken at each location. Points are coloured according to Contracting Party.
Confidence scoring:
A confidence scoring methodology, based on an approach developed by ICG-Eut to validate the output from their COMPEAT Tool, was applied to evaluate the robustness of reported trends for each plankton dataset for each assessment unit it intersected. For each assessment unit or fixed-point station temporal confidence was evaluated using two metrics, which are referred to here as specific temporal confidence (STC) and general temporal confidence (GTC).
STC was calculated on an annual basis as the proportion of months when samples were reported out of a total of 12 possible months. For example, if a dataset reported samples within an assessment unit only for June, July and August in a particular year, the STC for that year would be 0,25. GTC was calculated as the proportion of unique months with recorded samples out of the total number of potential months across the time-series. For example, a dataset commencing in January 2015 and ending in December 2019 contains a total of 60 potential months. If samples were reported for 30 of those months, the GTC would be 0,5. An overall temporal confidence score was then determined by calculating both the mean STC across all years and subsequently the mean of this value with the GTC.
Spatial confidence was also evaluated for distributed datasets such as the CPR. However, for fixed-point stations spatial confidence was not considered since there was no spatial element to the data. For each COMP4 assessment unit spatial confidence was calculated using two metrics, which similarly are referred to here as specific spatial confidence (SSC) and general spatial confidence (GSC).
SSC was calculated on an annual basis based on the proportion of longitudinal and latitudinal ranges of an assessment unit covered by the spatial extent of samples. For example, if an assessment unit spanned longitude from 1 to 5° E, and the distribution of samples collected within that assessment unit within a particular year ranged from 2 to 4° E, the proportion of longitudinal range covered by samples would be 0.5. The same procedure is also applied to the latitudinal range. The SSC for a particular year would be the mean of the longitudinal and latitudinal proportions covered by the spatial extent of samples.
GSC was derived using the same approach as SSC, but across all samples in a dataset that intersect with an assessment unit. An overall spatial confidence score was then determined by calculating the mean SSC across all years and subsequently calculating the mean of this value with the GSC.
Finally, an overall confidence score was calculated as the mean of temporal and spatial confidence. For fixed-point stations, spatial confidence was not considered, therefore only temporal confidence was used to represent the overall confidence of fixed-point station data.
Lifeform construction:
The eight plankton lifeforms highlighted in this assessment were chosen due to their ecological relevance and owing to the high confidence in their classification (Table d). All lifeforms were defined based on common ecological traits. The rationale for selecting the lifeforms and additional criteria containing supplementary information on lifeforms is listed in Table e.
Lifeform | Confidence | Reason for confidence (where not ‘high’) |
---|---|---|
(micro)Phytoplankton | High | |
Large microphytoplankton | Medium | Can reliably identify individual plankton species size class but cannot always reliably assign the size trait if the group counted spans taxa that are both larger and smaller than 20 microns. |
Small microphytoplankton | Medium | |
Diatoms | High | |
Dinoflagellates | High | |
Autotrophic and mixotrophic dinoflagellates | Medium | Can identify taxa, but assigning feeding mechanism trait is not always clear (see e.g.,: discussion in Flynn et al., (2019)) |
Pelagic diatoms | High | |
Tychopelagic diatoms | High | |
Potentially toxic and nuisance diatoms | Low | Designation of some algal blooms as “harmful” (i.e.,: Harmful Algal Blooms, ‘HABS’), relates more to societal assessment than plankton traits, these ‘lifeforms’ are therefore not currently recommended for use though they are defined in the traits list and will be the focus of future development work. Specific issues include: • The toxin producing diatom genus Pseudo-nitzschia contains both amnesic shellfish toxin-producers which can render shellfish unfit for human consumption and potentially negatively impact the health of marine mammals, and non-toxin-producing species/individuals. It is not possible to identify these cells to species level using routine light microscopy; some toxin and non-toxin producing species are morphologically identical. • The genus Alexandrium contains both paralytic shellfish toxin- and non-toxin-producing species/strains and it is not possible to distinguish these using routine light microscopy; some toxin and non-toxin producing species are morphologically identical. • The taxon Karenia mikimotoi forms high biomass blooms which strips the water column of oxygen which can be fatal to other lifeforms (Barnes et al., 2015). • Not all datasets included in PLET reliably record key species (e.g.: CPR does not record Alexandrium) |
Potentially toxic and nuisance dinoflagellates | Low | |
Ciliates | Low | • Ecological function can be duplicated by mixotrophic dinoflagellates. Ciliates do not preserve well in the standard 0,5% Lugol’s iodine preservative used to preserve phytoplankton samples and some (but not all) are too small to be well sampled by many of the datasets currently in PLET. |
Holoplankton | Medium | • May not identify taxa specifically enough to determine traits. • Some of the rarer species are resuspended from the seabed and definition of their holo- or meroplanktonic status is difficult |
Meroplankton | Medium | |
Gelatinous zooplankton | High | |
Fish larvae/eggs | High | |
Carnivorous zooplankton | Medium | Can identify taxa, but assigning diet trait is unclear, especially at different life stages. |
Non-carnivorous zooplankton | Medium | |
Crustaceans | High | |
Small copepods | High | |
Large copepods | High |
Lifeform | Traits | Criteria |
---|---|---|
Diatoms | 'Diatom' only | PhytoplanktonType=Diatom |
Dinoflagellates | 'Dinoflagellate' only | PhytoplanktonType=Dinoflagellate |
Gelatinous zooplankton | 'Gelatinous' only | PlanktonType=Zooplankton AND Gelatinous=Y |
Fish larvae/eggs | 'Fish' only | ZooType=Fish |
Carnivorous zooplankton | 'Carnivore' only | PlanktonType=Zooplankton AND Diet=Carnivore |
Non-carnivorous zooplankton | 'Zooplankton' AND either 'Herbivore', 'Omnivore', OR 'Ambiguous' | PlanktonType=Zooplankton AND (Diet=Herbivore OR Omnivore OR Ambiguous) |
Crustaceans | 'Crustacean' only | Crustacean=Y |
Large phytoplankton | 'Phytoplankton' AND 'Lg' | PlanktonType=Phytoplankton AND PhytoplanktonSize=Lg |
Small phytoplankton | 'Phytoplankton' AND 'Sm' | PlanktonType=Phytoplankton AND PhytoplanktonSize=Sm |
Phytoplankton | 'Phytoplankton' only | PlanktonType=Phytoplankton |
Autotrophic and mixotrophic dinoflagellates | 'Dinoflagellate' AND either 'Auto' OR 'Auto/Mixo' | PhytoplanktonType=Dinoflagellate AND (FeedingMech=Auto OR Auto/Mixo) |
Pelagic diatoms | 'Diatom' AND 'Pelagic' | PhytoplanktonType=Diatom AND DiatomDepth=Pelagic |
Tychopelagic diatoms | 'Diatom' AND 'Tychopelagic' | PhytoplanktonType=Diatom AND DiatomDepth=Tychopelagic |
Nuisance and toxin-producing diatoms | 'Diatom' AND either 'Toxic' OR 'Nuisance' | PhytoplanktonType=Diatom AND (HAB = Toxic) |
Nuisance and toxin-producing dinoflagellates | 'Dinoflagellate' AND either 'Toxic' OR 'Nuisance' | PhytoplanktonType=Dinoflagellate AND (HAB = Toxic) |
Holoplankton | 'Holoplankton' only | Habitat=Holoplankton |
Meroplankton | 'Meroplankton' only | Habitat=Meroplankton |
Large copepods | 'Copepod' AND 'Lg' | Copepod=Y AND ZooSize=Lg |
Small copepods | 'Copepod' AND 'Sm' | Copepod=Y AND ZooSize=Sm |
Ciliates | 'Ciliate' only | PhytoplanktonType=Ciliate |
Microflagellates | 'Dinoflagellate' AND 'Sm' | PhytoplanktonType=Dinoflagellate AND PhytoplanktonSize=Sm |
The database master species list (Ostle et al., 2021) was built by assigning functional traits to each species for a dataset and then adding additional new datasets to expand the master species list (Figure e). The species list for each new dataset was assigned a unique Aphia ID via the World Register of Marine Species (WoRMS). The new dataset’s species list was then compared with the plankton database’s master species list via Aphia IDs and any new species were identified. This process ensures that each species is only entered in the database once. The new species were then manually assigned functional traits by searching the literature; fields were left blank where functional traits for species were unknown. Once traits were assigned, the new species were added to the master species list. Queries were constructed (Table e) to build lifeforms from the functional trait information (McQuatters-Gollop et al., 2019).
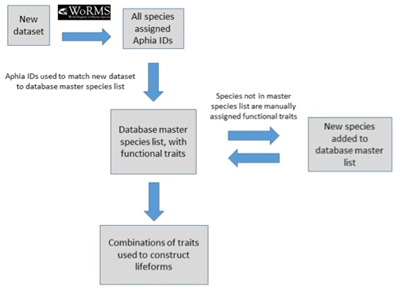
Figure e: Schematic illustrating the process undertaken to assign functional traits to species, then species to lifeforms. Each species must first be assigned a unique Aphia ID to determine whether it is already present in the master species list (McQuatters-Gollop et al., 2019).
A simple method of confidence assessment was applied for each lifeform (Table f). Using expert opinion, each lifeform was evaluated on two characteristics: the ability to identify and speciate organisms in that lifeform using light microscopy and the understanding of the accuracy of determining traits assigned to the lifeform. For example, medium confidence is assigned to the lifeforms ‘autotrophic dinoflagellates’ and ‘mixotrophic dinoflagellates’ because the mode of nutrition of many dinoflagellate species is currently uncertain (Flynn et al., 2019). Thus, the accuracy of assigning the lifeform category is medium. Likewise, the lifeform ‘non-carnivorous zooplankton’ has a medium confidence designation since the feeding habits of many abundant and common zooplankton species remain not strictly defined. Further investigation must also be conducted to decide whether both harmful-bloom-forming algae and potentially toxin-producing lifeforms should be considered in future assessments of this indicator. Work is ongoing to increase confidence in lifeforms, however, this work is resource dependent.
Easy to ID/speciate | Difficult to ID/speciate | |
---|---|---|
Known traits | High | Low |
Unknown traits | Low | Low |
Assessing links to pressures:
The Boruta algorithm (Kursa and Rudnicki, 2010) was applied to evaluate which environmental variables were the best predictors of lifeform abundance. The Boruta algorithm is a tree-based permutational variable importance method which uses a wrapper around random forest to evaluate the predictive performance of each variable being tested against a shuffled set of the same predictor variables. If a variable achieves better predictive accuracy than the highest-performing shuffled variable, it is determined to be important and is assigned a score based on the mean decrease in predictive accuracy when the variable is excluded from the model.
For each unique combination of dataset, assessment unit, and lifeform whenever a significant Kendall statistic (i.e., a significant change in lifeform abundance over the time-period assessed) was observed, a separate permutational variable importance process based on random forest (Boruta algorithm) was conducted to evaluate relationships between lifeform abundance and a set of 16 environmental variables described in Table g. Random forest is robust to detecting separate effects from collinear predictors, since it uses an ensemble approach based on agreement from many trees which each test a different subset of the predictor variables. Based on the results of the previous assessment, a table of implicating factors was developed, linking lifeforms with a set of environmental pressures with plausible links to variation in lifeform abundance (Table h). Only variables indicated in Table h were tested for each lifeform.
Table g: Environmental variables and their descriptions and sources evaluated in the random forest permutational variable importance process.
Variable name | Variable description | Source |
---|---|---|
Sea surface temperature | Temperature of surface layer, as measured by satellite | International Comprehensive Ocean-Atmosphere Data Set (ICOADS): https://psl.noaa.gov/data/gridded/data.coads.1deg.html |
Salinity | Salinity of the surface layer | European Regional Seas Ecosystem Model (ERSEM; NWSHELF_MULTIYEAR_PHY_004_009): https://doi.org/10.48670/moi-00059); |
Total oxidised nitrogen | Total oxidised nitrogen concentration in surface layer | In situ data from Marine Scotland Science (MSS): https://doi.org/10.7489/1881-1 and Aarhus University (Svendsen et al., 2005) |
Nitrate | Nitrate concentration in surface layer | European Regional Seas Ecosystem Model (ERSEM; NWSHELF_MULTIYEAR_BGC_004_011): https://doi.org/10.48670/moi-00058); In situ data from Plymouth Marine Laboratory (PML): https://www.westernchannelobservatory.org.uk/l4_nutrients.php |
Phosphate | Dissolved inorganic phosphate concentration in surface layer | European Regional Seas Ecosystem Model (ERSEM; NWSHELF_MULTIYEAR_BGC_004_011; https://doi.org/10.48670/moi-00058); In situ data from Plymouth Marine Laboratory (PML): https://www.westernchannelobservatory.org.uk/l4_nutrients.php and Marine Scotland Science (MSS): https://doi.org/10.7489/1881-1 |
Total phosphorous | Total dissolved phosphorous concentration in surface layer | In situ data from Aarhus University (Svendsen et al., 2005) |
N:P ratio | The ratio of molar nitrogen concentration to molar phosphorous concentration | Calculated from European Regional Seas Ecosystem Model (ERSEM; NWSHELF_MULTIYEAR_BGC_004_011): https://doi.org/10.48670/moi-00058); In situ data from Plymouth Marine Laboratory (PML): https://www.westernchannelobservatory.org.uk/l4_nutrients.php, Marine Scotland Science (MSS): https://doi.org/10.7489/1881-1 and Aarhus University (Svendsen et al. 2005) |
Silicate | Dissolved silicate concentration in surface layer | In situ data from Marine Scotland Science (MSS): https://doi.org/10.7489/1881-1, Plymouth Marine Laboratory (PML): https://www.westernchannelobservatory.org.uk/l4_nutrients.php and Aarhus University (Svendsen et al., 2005) |
Wind speed | Wind speed (a proxy for turbulence) | International Comprehensive Ocean-Atmosphere Data Set (ICOADS); https://psl.noaa.gov/data/gridded/data.coads.1deg.html |
Mixed layer depth | The depth below the surface where the steepest change in density occurs (the thickness of the surface layer in which photosynthesis can occur) | European Regional Seas Ecosystem Model (ERSEM; NWSHELF_MULTIYEAR_PHY_004_009): https://doi.org/10.48670/moi-00059) |
Light attenuation | The extinction coefficient for visible light, or the decrease in the intensity of solar radiation with depth (a proxy for the opacity of the water column) | European Regional Seas Ecosystem Model (ERSEM): NWSHELF_MULTIYEAR_BGC_004_011; https://doi.org/10.48670/moi-00058) |
Precipitation | Rate of precipitation (a proxy for freshwater input) | International Comprehensive Ocean-Atmosphere Data Set (ICOADS); https://psl.noaa.gov/data/gridded/data.coads.1deg.html |
Current velocity | Current velocity in surface layer (a proxy for horizontal transport which affects how quickly organisms are advected to adjacent systems) | European Regional Seas Ecosystem Model (ERSEM; NWSHELF_MULTIYEAR_PHY_004_009; https://doi.org/10.48670/moi-00059) |
Atlantic Multidecadal Oscillation (AMO) | The Atlantic Multidecadal Oscillation, also known as Atlantic Multidecadal Variability, is the theorised variability of the sea surface temperature of the North Atlantic Ocean on the timescale of several decades | National Oceanic and Atmospheric Administration (NOAA); https://psl.noaa.gov/data/timeseries/AMO/ |
North Atlantic Oscillation (NAO) | The North Atlantic Oscillation is a weather phenomenon over the North Atlantic Ocean of fluctuations in the difference of atmospheric pressure at sea level between the Icelandic Low and the Azores High | National Oceanic and Atmospheric Administration (NOAA): https://www.ncdc.noaa.gov/teleconnections/nao/ |
pH | Sea water pH reported on total scale | European Regional Seas Ecosystem Model (ERSEM; NWSHELF_MULTIYEAR_BGC_004_011): https://doi.org/10.48670/moi-00058) |
Variable in Figure 3 | Variable name | Diatoms | Dinoflagellates | Holoplankton | Meroplankton | Large copepods | Small copepods | Fish larvae/eggs | Gelatinous |
---|---|---|---|---|---|---|---|---|---|
Temperature | Sea surface temperature | ✓ | ✓ | ✓ | ✓ | ✓ | ✓ | ✓ | ✓ |
Salinity | Salinity | ✓ | ✓ | ✓ | ✓ | ✓ | ✓ | ||
Nutrients | Total nitrogen | ✓ | ✓ | ||||||
Nitrate | ✓ | ✓ | |||||||
N:P ratio | ✓ | ✓ | |||||||
Phosphate | ✓ | ✓ | |||||||
Total phosphorous | ✓ | ✓ | |||||||
Silicate | ✓ | ✓ | |||||||
Wind | Wind speed | ✓ | ✓ | ✓ | ✓ | ✓ | ✓ | ||
Mixed layer depth | Mixed layer depth | ✓ | ✓ | ✓ | ✓ | ✓ | ✓ | ||
Attenuation | Light attenuation | ✓ | ✓ | ✓ | ✓ | ||||
Precipitation | Precipitation | ✓ | ✓ | ✓ | ✓ | ✓ | ✓ | ✓ | ✓ |
Current | Current velocity | ✓ | ✓ | ✓ | ✓ | ✓ | ✓ | ✓ | ✓ |
Climate index | Atlantic Multidecadal Oscillation | ✓ | ✓ | ✓ | ✓ | ✓ | ✓ | ✓ | ✓ |
North Atlantic Oscillation | ✓ | ✓ | ✓ | ✓ | ✓ | ✓ | ✓ | ✓ | |
pH | pH | ✓ | ✓ | ✓ | ✓ |
Prior to any manipulation of data, lifeform time-series were divided into separate training and testing sets. The training data were used for variable selection and for generating the random forest models, while the testing data were used to validate model predictive accuracy. For the purposes of this analysis, the training data were limited to all months prior to the assessment period (e.g., 1960 to 2014 for CPR data) and the testing data were restricted to the assessment period itself (e.g., 2015 to 2019).
This analysis covered the full temporal extent of each plankton dataset (1960 to 2019 in the case of CPR data). While gridded data from NOAA were available from 1960 to 2019, the longest duration modelled dataset for nutrients only spanned from 1993 to 2019, with in-situ nutrient datasets commencing as early as 1980. To evaluate long-term links to pressures and avoid excluding the first several decades of many plankton time-series from our analysis the ‘missRanger’ package for R (Mayer and Mayer, 2019) was used to perform multiple imputation by chained random forests. This method uses multiple random forest regressions to impute missing values based on collinearities among observed values in the predictors. For each variable containing missing values the algorithm generates a separate regression model based on all the other predictors. To improve imputation performance, a numeric variable representing ‘month’ was included in this step to better predict the consistent seasonal patterns in some variables. Missing values in the predictors were imputed separately for the training and testing datasets. It is important to note that no imputation of lifeform abundance time-series was performed to avoid introducing greater uncertainty into the process of linking to pressures.
All variables including lifeform abundance were smoothed to remove seasonality and uncover long-term trends by calculating the mean value across a 12 month moving window (Figure f). This step required the first and last six months of data from training and testing sets to be excluded from the analysis.
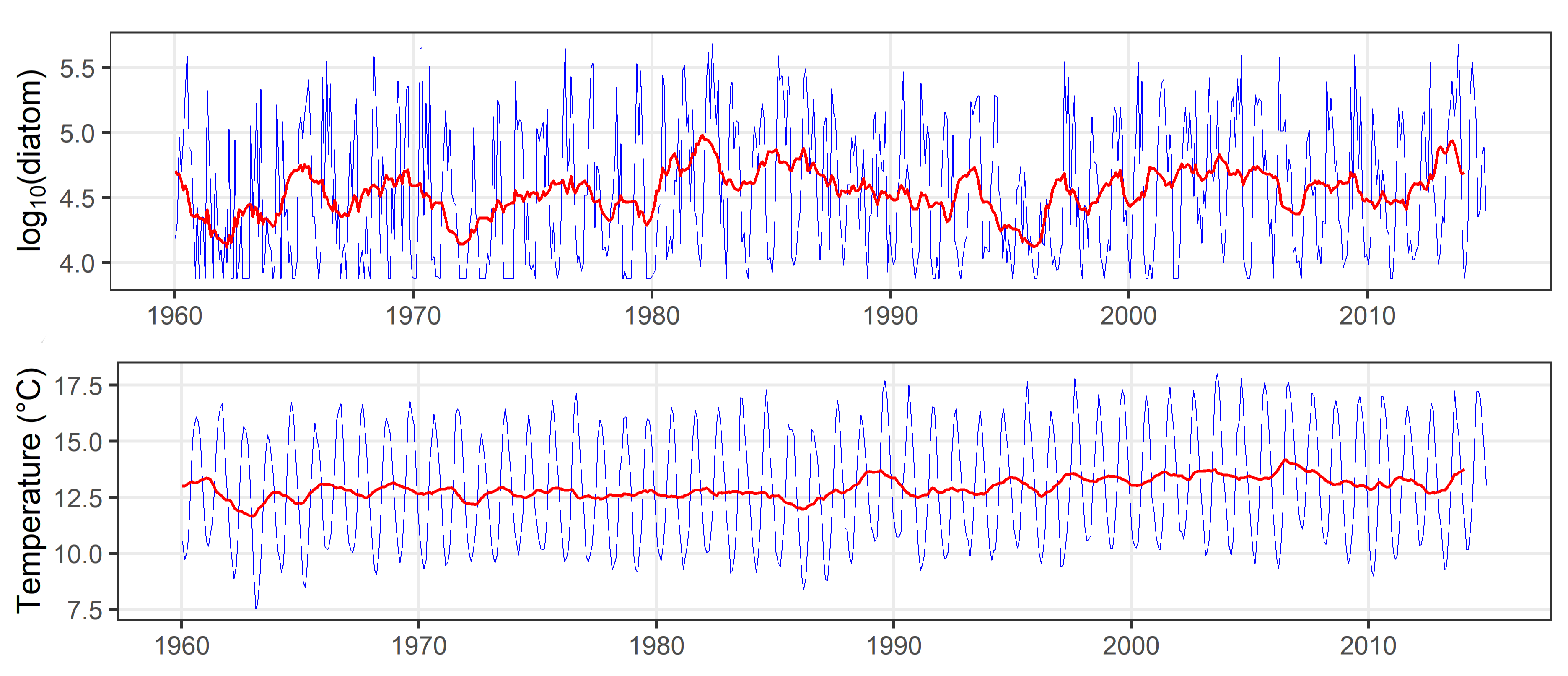
Figure f: Long-term time-series (1960 to 2019) for diatom abundance from CPR, and sea surface temperature from ICOADS, for the ‘Channel well mixed’ COMP4 assessment unit. Each plot displays monthly mean values (blue line) and 12-month moving average or monthly long-term trend (red line) in each time-series.
Values for each variable were calculated as the mean of monthly mean gridded values (modelled and remotely sensed; Table g) intersecting each COMP4 assessment unit. For fixed-point stations, mean values were calculated from all measurements within a 5-nautical mile radius of the station. Where in-situ data were available (total nitrogen, nitrate, phosphate, total phosphorous, silicate) they were evaluated instead of the modelled environmental variables. For Atlantic Multidecadal Oscillation (AMO) and North Atlantic Oscillation (NAO), monthly values were applied identically across all assessment units since these variables have basin-scale influence likely to cover most of the assessment region.
Environmental variables were grouped into ten categories to simplify visualisation in map format (Table h). Categorisations of the most important predictor variable for each model, or the predictor variable which resulted in the greatest mean decrease in accuracy when it was removed, were reported across a map. Instances when the validation step with the testing data indicated significant positive correlation (p ≤ 0,05) between predicted and observed lifeform abundance values were also indicated in this map to provide greater confidence in the variable importance results and to avoid reporting spurious relationships.
It is important to note that observing high importance of any environmental variable is simply an indication that it co-varied with lifeform abundance. Our approach cannot imply causation since it is impossible to hold all other potential influences on lifeform abundance constant, as would be the case in a controlled experimental setting.
Integration of indicator results:
A primary objective of this indicator assessment was to integrate results to facilitate an understanding of changes occurring across pelagic habitat types within OSPAR Regions II, III and IV. This required indicator results for each OSPAR Region to be integrated according to the following pelagic habitat categories: variable salinity, coastal, shelf, and oceanic / beyond shelf. This categorisation of COMP4 assessment units and fixed-point stations is described in Table a and Table b. To meet this objective, the focus was on the primary direction of change detected across assessment units and fixed-point stations within each pelagic habitat category for each of the 8 high confidence plankton lifeforms highlighted in this assessment. The mean confidence, spatial representativeness were then reported, and most likely links to environmental pressures.
As an example, changes in dinoflagellate abundance were assessed across 4 COMP4 assessment units and 4 fixed-point stations representing variable salinity habitats within OSPAR Region II. If 1 decreasing trend, 4 increasing trends, and 3 instances of no trend were detected across these locations, an increasing net trend would be reported and the proportion of assessment units studied where this trend was detected, in this case 0,5.
To report the level of confidence in this result, the mean confidence score for locations where dinoflagellate abundance was increasing, was calculated. COMP4 assessment units and fixed-point stations as equivalent were considered for this integration.
To report the spatial representativeness of the result, the proportion of the total number of COMP4 assessment units considered in the analysis was calculated, in this case 8, out of the total number of possible COMP4 assessment units representing variable salinity habitats within the OSPAR Region, in this case 16. Therefore, the spatial representativeness of the result would be 0,5. Note that fixed-point station datasets do not contribute to this score.
Finally, to report links to environmental pressures which can drive changes in lifeform abundance for the net trend, environmental variables for each location based on their relative variable importance were ranked, with 1 assigned to the variable with highest importance, 2 to the variable with second highest importance and so on. For locations where the net trend was increasing, the mean rank of each environmental variable was calculated and the variable with the lowest mean rank reported.
Assessing the status of pelagic habitats:
To assign a designation of assessment status to pelagic habitats based on the integration of indicator results, a semi-quantitative methodology described in McQuatters-Gollop et al., (2022) was applied, which was developed from the lessons gained from the previous OSPAR assessment (IA 2017). In this case, the status of a habitat type can be designated as either “Good”, “Unknown”, “Not good”, or “Not assessed” (Table i). Following the criteria outlined in this study, if a pelagic habitat has been assessed, it should by default be considered as either “Unknown” or “Not Good”. At this stage it is not realistic to assign “Good” status to pelagic habitats, since it is difficult to develop meaningful assessment thresholds for plankton and generally not possible to determine whether a particular state is desirable or undesirable, except under specific circumstances such as eutrophication. Following this logic, the status of pelagic habitats should be considered “Unknown” by default. In cases when change has been detected and that change can be confidently linked to the impact of an anthropogenic pressure, the status of this habitat is “Not good”.
Table i: Biodiversity status categories and colours used for the interpretation, by expert judgement, of indicator biodiversity state (McQuatters-Gollop et al., 2022).
Not good | Indicator value is below assessment threshold, or change in indicator represents a declining state, or indicator change is linked to increasing impact of anthropogenic pressures (including climate change), or indicator shows no change but state is considered unsatisfactory |
Unknown | No assessment threshold and/or unclear if change represents declining or improving state, or indicator shows no change but uncertain if state represented is satisfactory |
Good | Indicator value is above assessment threshold, or indicator represents improving state, or indicator shows no change but state is satisfactory |
Not assessed | Indicator was not assessed in a region due to lack of data, lack of expert resource, or lack of policy support. |
The status at the level of each of the 8 high confidence lifeforms within each pelagic habitat within OSPAR Regions was considered and the results integrated for multiple lifeforms to assign a single quality status designation for the pelagic habitat type. For the status of a lifeform to be shifted from “Unknown” to “Not good” the results of the integration had to meet certain criteria:
- The net trend should be either increasing or decreasing and must be present in at least 0,5 of the locations assessed.
- The mean confidence for locations considered for the determination of the net trend must be at least 0,5.
- The spatial representativeness of the assessed locations out of the total number of possible locations for that habitat type must be at least 0,5.
- The environmental pressure with the greatest mean rank for locations expressing the net trend must represent either sea surface temperature, pH, or nutrients.
- The mean rank of the most important environmental pressure must be ≤ 3, indicating that across all locations the variable ranks in the top 3 most important for predicting the abundance of the lifeform.
If all the above criteria are met, the lifeform is assigned a status of “Not good”. If 25% or more of assessed lifeforms within a pelagic habitat type are assigned a status of “Not good” then the whole habitat type is also assigned a status of “Not good”. Otherwise, the habitat type is assigned a status of “Unknown”.
Lifeform pairs indicator approach:
Although this assessment focused primarily on long-term trends in lifeform abundance due to their stronger links with environmental pressures at large spatial scales, the lifeform pairs indicator was also evaluated for the current assessment (which constitutes the PH1 indicator as originally defined in IA2017).
Tett et al., (2008) proposed to track changes in the state of the plankton community by means of plots in a state space and calculating a Plankton Community Index, referred to here as a Plankton Index (PI). The conceptual framework is that ecosystems can be viewed as systems with an instantaneous state defined by values of a set of system state variables which are attributes of the system that change with time in response to each other and external conditions. Building on this approach and plotting plankton lifeform abundance in a multi-dimensional state space provides a means of monitoring changes in the structure of plankton communities. A state can be defined as a single point in state space, with coordinates provided by the values of the set of state variables, in this case two lifeform abundances, which together are used as a pair.
The plankton community index is calculated by initially mapping relative plankton lifeform abundances from an assessment period in a state space. The distribution of lifeform abundances within the state space is then used to define an envelope, representing the prevailing conditions for the assessment period. Finally, a set of abundance values from a comparison period are mapped onto the state space to evaluate the plankton community index, which represents the proportional similarity of the two periods.
Mapping lifeforms in a state space
In the example illustrated in Figure g for diatoms and dinoflagellates, the axes of the two-dimensional space are the abundance values of the two lifeforms. Each point represents the state of the ecosystem in terms of the two lifeforms at the time of sample collection. Subsequent samples yield additional pairs of abundance values that can be mapped onto the lifeform state space.
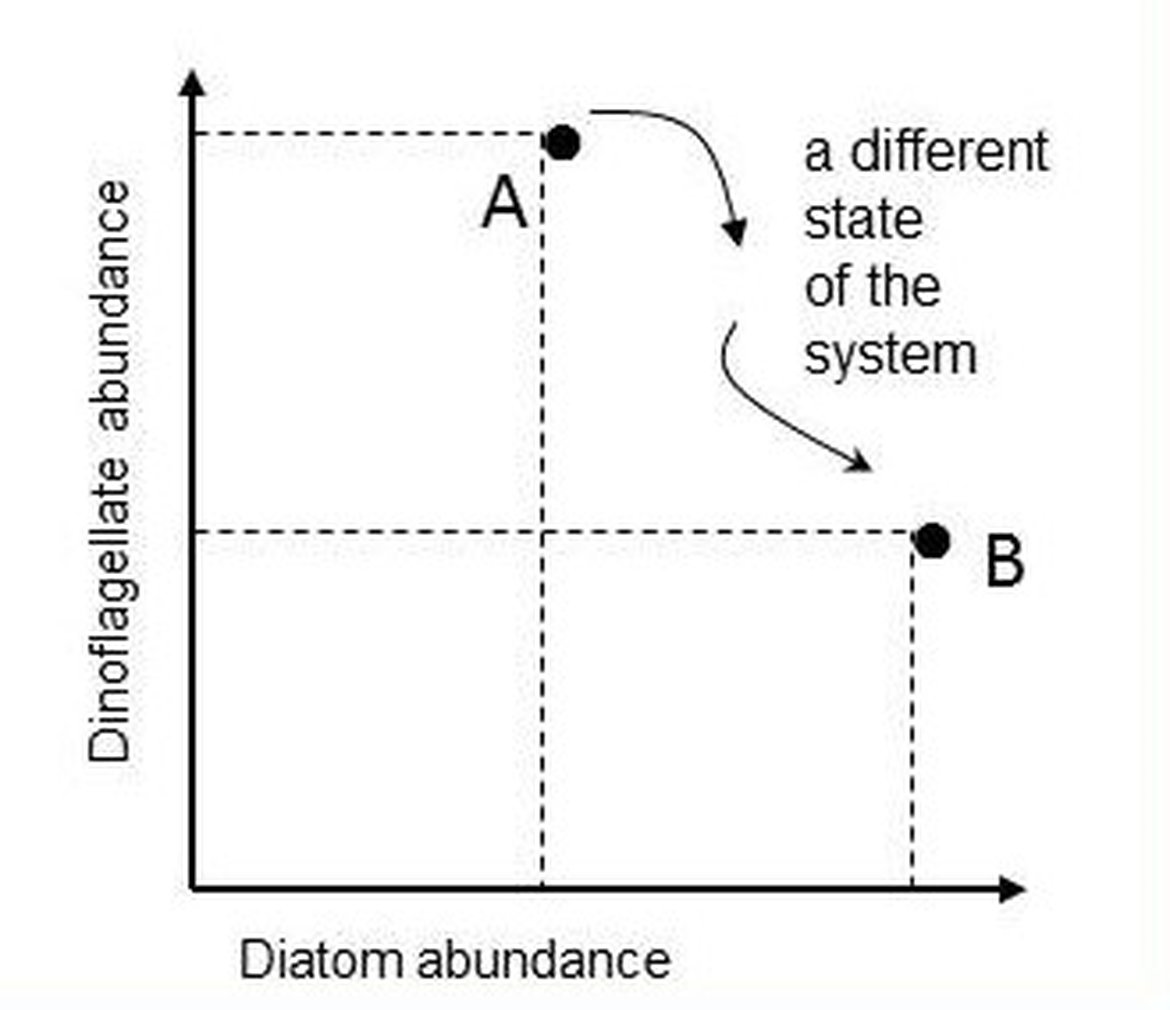
Figure g: Mapping the abundance of two lifeforms in state space
Point A is the ecosystem state at the instant a water sample was taken and is characterised by the abundance values of two lifeforms. Another sample, taken in the same location, yields abundance values that map to a different point in the diatom-dinoflagellate state space (point B).
The path between the two states is called a trajectory, and the plankton condition is defined by the trajectory drawn in the state space by a set of points. Such trajectories reflect: (i) cyclic and medium-term variability (the higher order consistencies in the plankton that result from seasonal cycles, species succession and interannual variability); and (ii) long-term variability that might result from environmental pressure. The seasonal nature of plankton production and the succession of species in seasonally stratifying seas, result in this trajectory tending in a certain direction (as plankton growth increases in spring and declines during autumn), such that the trajectory tends towards its starting point. Given roughly constant external pressures, the data collected from a particular location over a period of years form a cloud of points in state space that can be referred to as a regime. Long-term variability may show a persistent trend of movement away from a starting point in state space.
Approach for defining the envelope
To define a regime, an envelope can be drawn about this group of points using a convex hull method. Because of theoretical arguments that the envelope should be doughnut-shaped with a central hole (Tett et al., 2008), bounding curves can be fitted outside and inside the cloud of points (Figure h). The data are from the CPR dataset for the ‘Channel well mixed’ COMP4 assessment unit. The colour of each point corresponds to the season within which it was sampled; blue = winter, green = spring, yellow = summer, red = autumn.
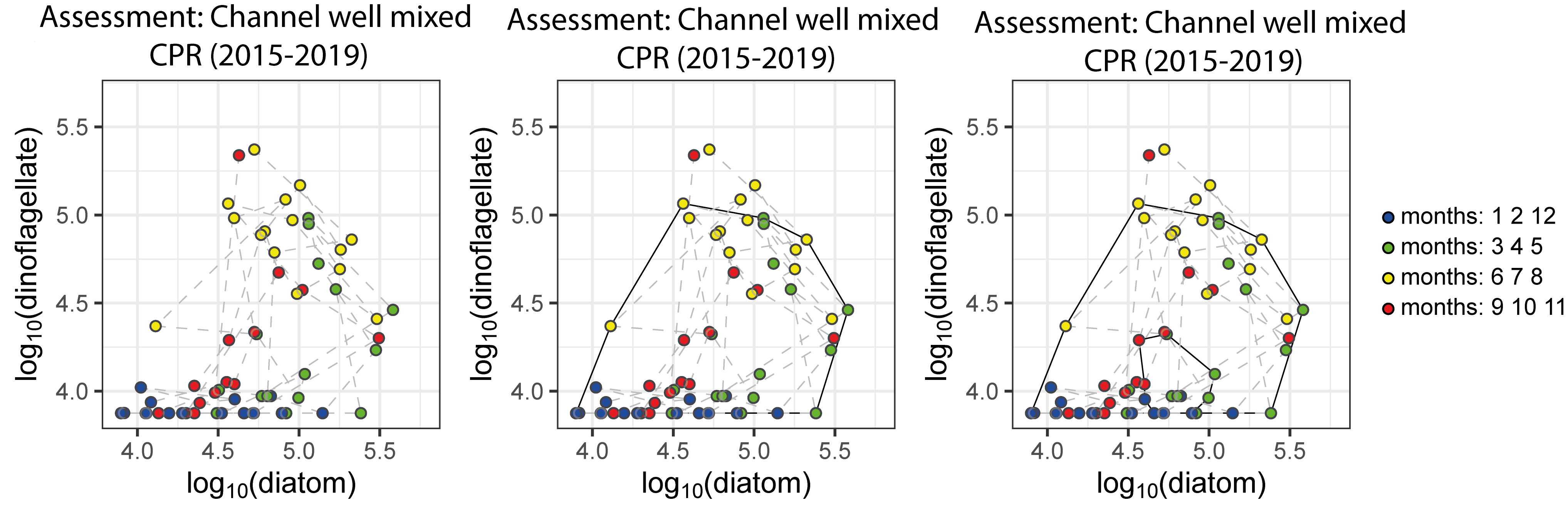
Figure h: Creating the envelope in three steps, from left to right: An example of a regime defined by the envelope drawn by the convex hull method
The size and shape of the envelope are sensitive to sampling frequency and the total number of samples. Envelopes are made larger by including extreme outer or inner points, and the larger the envelope, the less sensitive it will be to change in the distribution of points in state space and therefore to detect a change in condition. Conversely, if too many points are excluded the envelope will be small and even minor changes will result in a statistically significant difference. It is therefore desirable to exclude a proportion (p) of points, to eliminate these extremes, and so the 90-percentile was used. Envelopes are therefore drawn around the cloud of points to include a proportion (p=0,9) of the points, excluding the 5% of points that were most distant from the cloud's centre and the 5% of points that were closest to the centre.
For a Plankton Index to be calculated, it is necessary to establish a set of conditions as the basis for making comparisons. For this assessment the Plankton Index was calculated by using the assessment period (2015-2019) to define the envelope. For each dataset, all measurements collected prior to the assessment period were used for comparison. For the CPR data this meant the envelope was defined with samples from 2015 to 2019 and samples from 1960 to 2014 were used for comparison. This made it possible to maintain the same range of years to define the envelope across multiple datasets with different temporal coverage. Like the Kendall statistic, the PI was calculated independently for each combination of dataset, assessment unit and lifeform pair.
In the example shown in Figure i, CPR data collected from the ‘Channel well mixed’ COMP4 assessment unit during the assessment period (2015-2019), were used to create an envelope. The envelope, thus drawn (Figure i) defines a domain in state space that contains a set of trajectories of the diatom-dinoflagellate component of the pelagic ecosystem.
Calculating the plankton community index
The next step is to map a new set of data onto the same state space for comparison (Figure i). The value of the PI is the proportion of new points that fall between the inner and outer envelopes. In this example, 30% or 198 of the 660 new points lie outside, and the PI is 0,7. A value of 1,0 would indicate no change, and a value of zero would show complete change. The envelope was made by excluding 10% of points, so 66 (10% of 660) points are expected to fall outside. The exact probability of getting 198 by chance alone when only 66 are expected, can be calculated using a chi-square calculation (with 1 df and a 1-tail test). The value of 0,7 is significantly less than the expected value of 0,9, and so the difference between the two periods is statistically significant (p < 0,01).
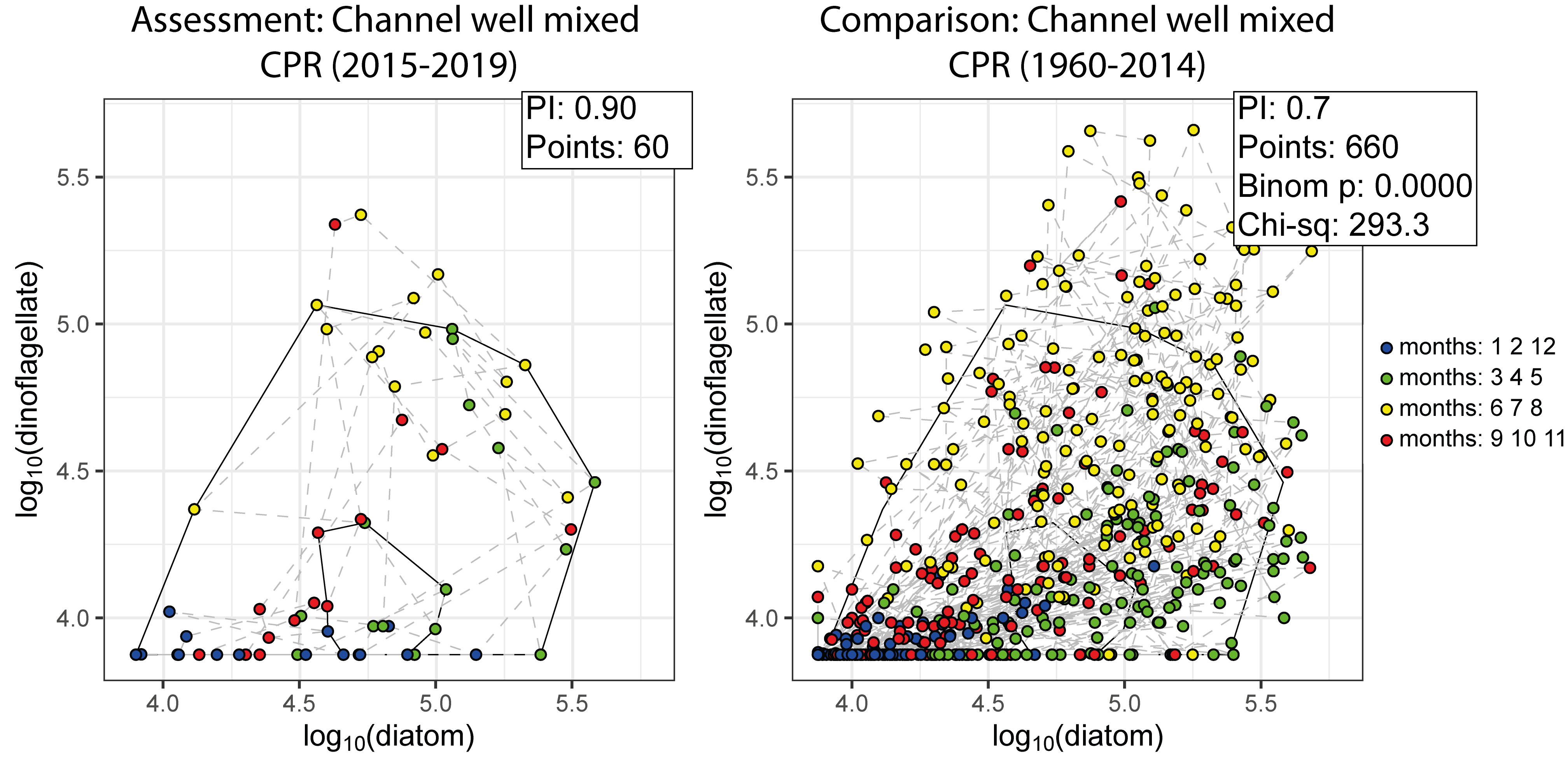
Figure i: An example for the comparison between assessment and comparison periods within the ‘Channel well mixed’ COMP4 assessment unit, with representing the assessment period of 2015-2019 and representing the comparison period of 1960-2014.
Résultats
Les tendances à long terme (1960-2019 ; Figure 2) indiquent que la plupart des formes de vie du plancton, y compris les diatomées, les dinoflagellés, l'holoplancton, les larves/œufs de poisson et les copépodes de grande taille (adultes ≥2 mm) et de petite taille (adultes <2 mm) voient leur abondance diminuer dans l'ensemble de l'Atlantique du Nord-Est. Les schémas spatiaux de la direction du changement pour les petits et les grands copépodes correspondent étroitement à ceux des diatomées. À l'inverse, le méroplancton présente une tendance à l'augmentation de l'abondance ou à l'absence de changement dans toutes les unités d'évaluation et dans toutes les stations à point fixe sauf une. Les schémas évidents à partir des données CPR largement distribuées sont reflétés par les stations à point fixe des eaux de transition adjacentes, sauf dans le cas des dinoflagellés, qui montrent une tendance à la hausse dans les eaux de transition d'Écosse (MSS, Royaume-Uni) et d'Allemagne (NLWKN, Allemagne). Cette divergence peut être due à la mauvaise conservation des dinoflagellés athécés dans les échantillons CPR, qui sont mieux conservés dans les séries de données des stations à point fixe.
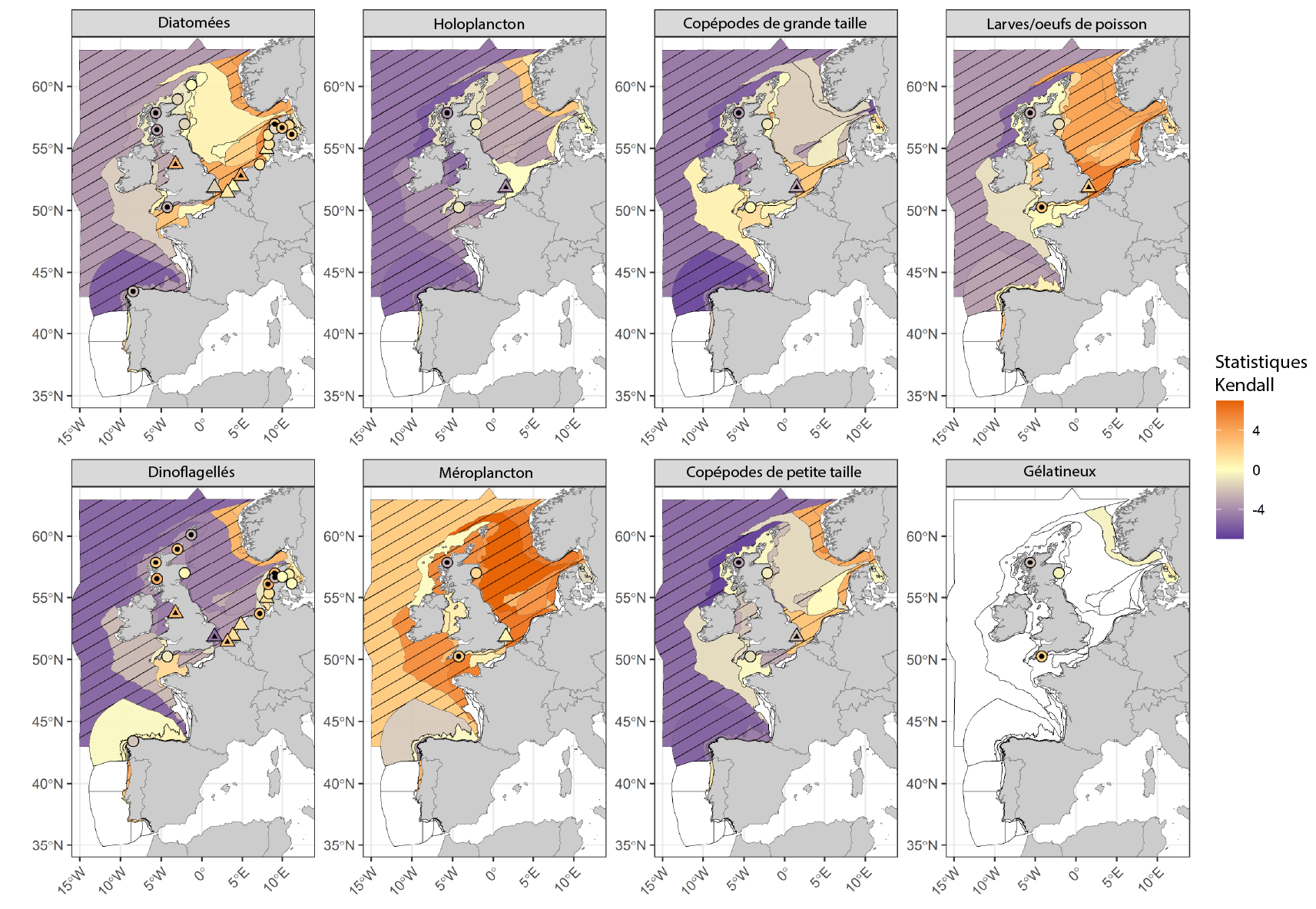
Figure 2 : Statistiques Kendall pour huit formes de vie planctoniques affichées sur les unités d'évaluation COMP4 et les stations à point fixe dans la Mer du Nord au sens large, les Mers celtiques, le golfe de Gascogne et la côte ibérique. Les panaches fluviaux sont représentés par des triangles. Les unités d'évaluation sont colorées en fonction des résultats du test de tendance de Kendall, qui indique l'ampleur de l'augmentation (> 0) et de la diminution (< 0) à long terme de l'abondance des formes de vie entre 1960 et 2019 (ou une période plus courte pour les stations fixes). Les unités d'évaluation, les stations à point fixe et les panaches fluviaux avec un symbole noir interne indiquent un changement statistiquement significatif (p ≤ 0,05). Les unités d'évaluation remplies en blanc indiquent que les données sont insuffisantes pour évaluer une tendance.
Au cours de la période d'évaluation actuelle (2015 à 2019), les données du CPR indiquent que l'abondance des dinoflagellés a continué à diminuer dans la mer du Nord septentrionale (voir la section « Results extended ») ; Figure j). L'holoplancton montre également des signes de déclin continu dans l'unité d'évaluation de la stratification saisonnière de l'Atlantique. Pour d'autres formes de vie et unités d'évaluation, les changements d'abondance conformes aux tendances à long terme n'étaient pas détectables au cours de la période d'évaluation de cinq ans ; toutefois, une période de cinq ans est probablement insuffisante pour une évaluation solide des changements environnementaux. Les nouvelles tendances apparues au cours de la période d'évaluation actuelle comprennent la diminution de l'abondance des grands copépodes dans l'unité d'évaluation "Stratification saisonnière de l'Atlantique", l'augmentation des larves/œufs de poisson dans la mer d'Écosse et les régions côtières du Royaume-Uni 1, et la diminution de l'abondance des diatomées à Norderney (NLWKN, Allemagne).
Les changements dans l'abondance des formes de vie ont été liés à la variation des pressions environnementales, agissant à la fois de manière externe (par exemple, les précipitations, la vitesse du vent) et interne (par exemple, le pH, la température de l'eau) sur le milieu marin. Nombre de ces pressions sont indirectement influencées par le changement climatique (Figure 3). Les résultats de la modélisation ont montré que l'augmentation des températures de surface de la mer était liée à la diminution de l'abondance des formes de vie planctoniques, en particulier des petits et grands copépodes dans l'Atlantique. Le changement de la température de l'eau était également fortement lié à l'augmentation de l'abondance du méroplancton dans la partie orientale de la mer du Nord. Les effets de l'augmentation de la température ont été le plus souvent détectés dans les habitats pélagiques du plateau continental et de l'océan. Si l'augmentation des températures peut avoir des effets directs, elle peut également être liée à une plus grande stratification et à la limitation des nutriments qui en résulte.
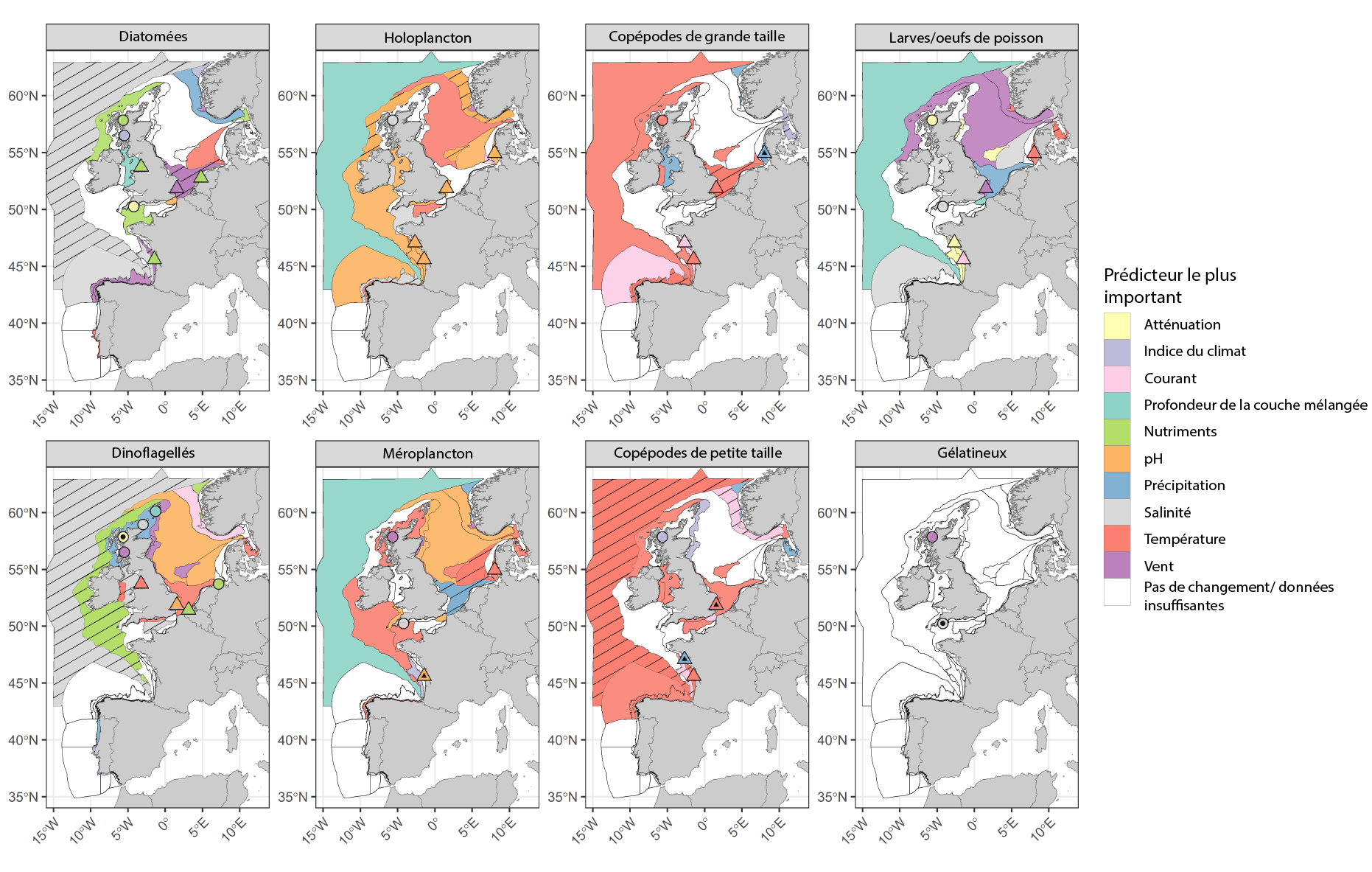
Figure 3 : Unités d'évaluation COMP4 pour huit formes de vie du plancton, colorées selon une catégorisation de la variable environnementale la plus importante pour prédire l'abondance de la forme de vie (voir la clé dans le tableau c). Les unités d'évaluation, les stations à point fixe et les panaches fluviaux avec un symbole noir interne indiquent une corrélation statistiquement significative entre les données d'essai prédites et observées, ce qui indique une plus grande fiabilité des résultats rapportés. Les unités d'évaluation remplies de blanc indiquent qu'il n'y a pas de changement dans l'abondance des formes de vie ou que les données sont insuffisantes pour évaluer une tendance. Les stations à point fixe et les panaches fluviaux ne sont affichés que lorsqu'une tendance dans l'abondance des formes de vie est présente.
Les liens avec la concentration de nutriments sont moins clairs, car il est difficile d'évaluer l'impact des concentrations de nutriments sur les formes de vie en raison de la période de latence entre l'apparition et l'absorption et l'assimilation par le phytoplancton. Toutefois, les résultats des modèles suggèrent qu'ils étaient plus apparents dans les régions côtières. Les changements dans l'abondance des diatomées et des dinoflagellés dans ces unités d'évaluation sont principalement liés à des augmentations du rapport N:P, entraînées par des réductions des concentrations de phosphore. Sur les 182 tendances significatives de l'abondance des formes de vie évaluées, 78 % n'indiquaient pas une capacité prédictive statistiquement significative des modèles basés sur les variables environnementales (c'est-à-dire p > 0,05).
Statistically significant continuation of long-term trends was only apparent in a few assessment units when the Kendall statistic was calculated for the assessment period only (2015 to 2019; Figure j), owing at least partially to the reduction in statistical power associated with detecting trends across such short time periods. Longer assessment periods increase the detection of many of the important changes which are occurring in plankton lifeforms, due to high inter-annual variability in lifeform abundance and gradual rates of change over time. Increasing the temporal extent also increases the detection of change because data from more historic periods are included (Bedford et al., 2020a; Bedford et al., 2020b)
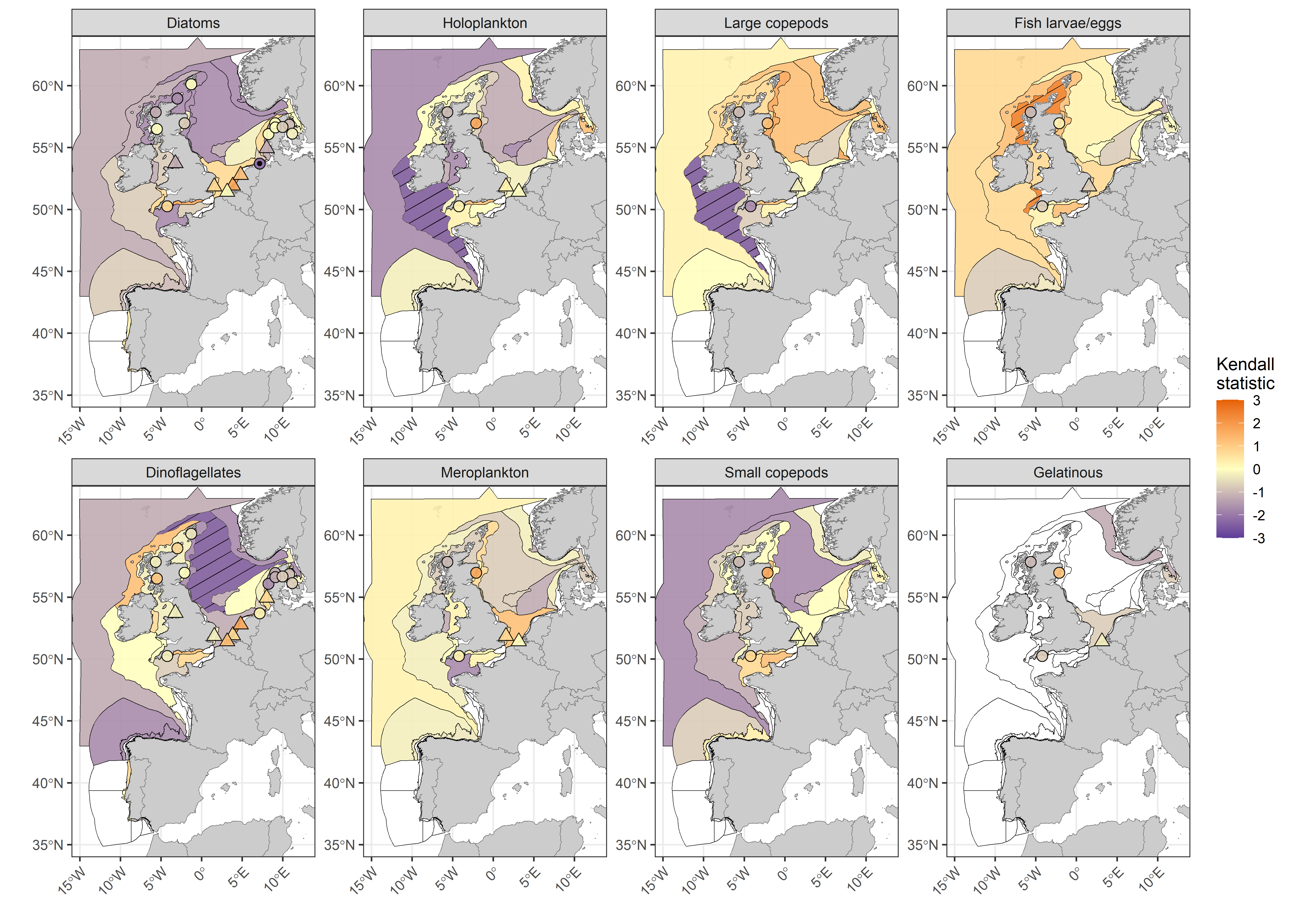
Figure j: Kendall statistics for eight plankton lifeforms displayed over COMP4 assessment units and fixed-point stations in the Greater North Sea, Celtic Seas, Bay of Biscay and Iberian Coast. River plumes are represented as triangles. Assessment units are coloured by the results of the Kendall trend test, which indicate the magnitude of increase (> 0) and decrease (< 0) in lifeform abundance from 2015-2019. Patterned assessment units, fixed-point stations and river plumes with an internal black symbol indicate statistically significant change (p ≤ 0,05). Assessment units filled in white indicate insufficient data to evaluate a trend.
The lifeform pairs indicator (PI), which indicates the degree of similarity in the co-abundance of lifeform pairs (Figure k) between the assessment period (2015 to 2019) and the comparison period (1960 to 2014) reveals that changes in diatoms and dinoflagellates were greatest in coastal waters surrounding the United Kingdom and within the Norwegian Trench, driven by reduced summer and autumn abundances of dinoflagellates. Diatoms and dinoflagellates showed the most stability within the Channel well mixed, Meuse and Elbe plume assessment units. Fixed-point stations, particularly those around Scotland, did not always reflect patterns in offshore CPR data. For gelatinous zooplankton and fish larvae/eggs there were insufficent records of gelatinous zooplankton to form any conclusions, however there was evidence of important changes in the Norwegian Trench and Kattegat Coastal assessment units. For holoplankton and meroplankton the greatest changes were along the Atlantic on the northern coast of Spain (Noratlantic Area NOR-NorO1) and in the Norwegian Trench. Widespread changes in the Dogger Bank, Eastern and Southern North Sea assessment units were caused by increasing trends in holoplankton winter abundance. Large and small copepods demonstrated a similar pattern to that of holoplankton and meroplankton. The PI also showed poor overlap with patterns in the Kendall statistic for individual lifeform abundances, which indicates there were important changes to seasonal trajectories which did not contribute to detectable trends in mean annual abundance.
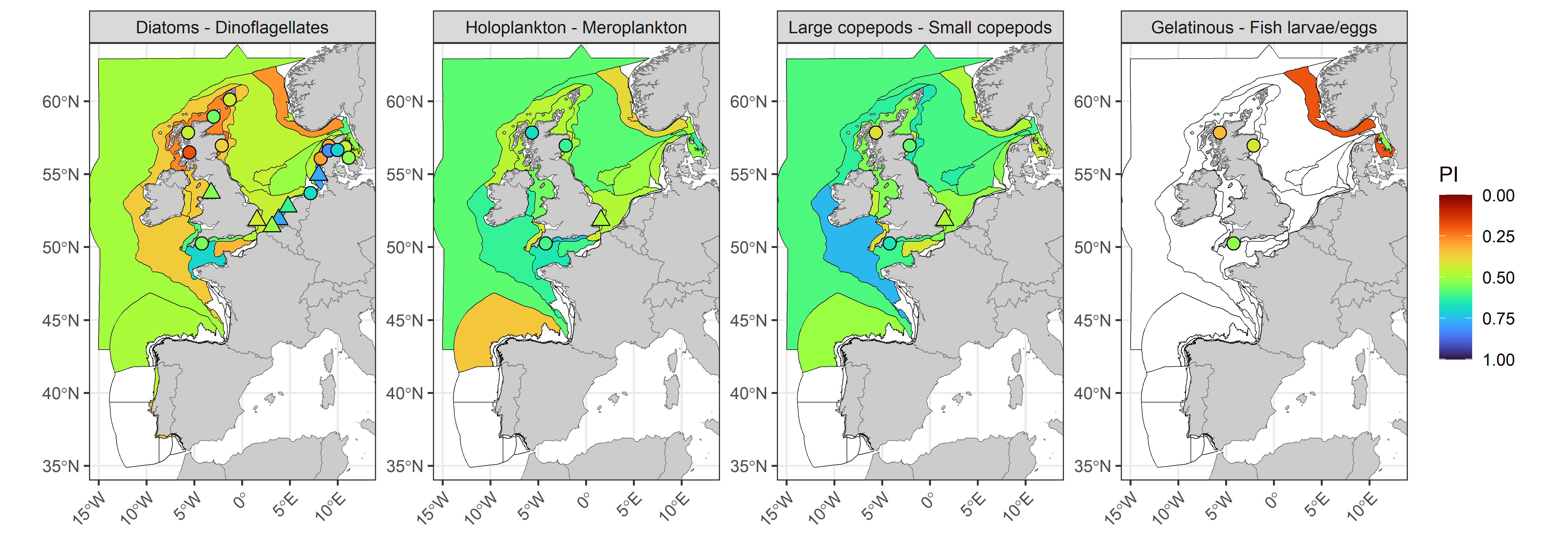
Figure k: Plankton lifeform pairs indicator (PI) for four lifeform pairs displayed over COMP4 assessment units and fixed-point stations in the Greater North Sea, Celtic Seas, Bay of Biscay and Iberian Coast. River plumes are represented as triangles. Assessment units are coloured according to the PI with an assessment period of 2015-2019 and a comparison period ranging from the beginning of each time-series up until the end of 2014. The PI indicates the proportion of months in the comparison period which fall within the assessment envelope. Assessment units filled in white indicate insufficient data to evaluate the PI.
The integration of the indicator results across pelagic habitat categories (Figure c) indicate that meroplankton in coastal and shelf areas of the Greater North Sea (Table j) are increasing in abundance and the increasing trends are closely linked to rising sea surface temperatures. Simultaneous declines in the abundance of holopankton in shelf regions have also been linked to temperature increase.
Table j: Integration of the indicator results for OSPAR Region II – Greater North Sea. Column names are described as follows: ↓: the number of COMP4 assessment units and fixed-point stations where decreasing trends have been detected, -: the number of COMP4 assessment units and fixed-point stations where no trends have been detected, ↑: the number of COMP4 assessment units and fixed-point stations where increasing trends have been detected, Dir: the net direction of change in the lifeform (↓: decreasing, ↑: increasing, -: stable), Trend: the percentage of assessment units exhibiting the respective trend, Conf: the mean confidence of datasets considered in the assessment, Change: a logical variable (TRUE/FALSE) to report whether a net trend is likely given the proportion of locations expressing the trend and the confidence and spatial represenativeness scores, Press1: the environmental pressure with the greatest mean rank for the respective trend, Rank1: the mean rank of the environmental pressure indicated under Pres1, nStn: the total number of fixed-point stations considered, totAssess: The total number of COMP4 assessment units and fixed-point stations considered, totCOMP4: The total number of potential COMP4 assessment units for the habitat category, spatialRep: the spatial representativeness score of the analysis. The status of the individual lifeforms are indicated by the colours in the Lifeform column, according to the legend in Table i. The overall status of the habitat category is indicated by the colour of the first column, which also identifies pelagic habitat types and follows the same colour legend.
Habitat | Lifeform | ↓ | - | ↑ | Dir | Trend | Conf | Change | Press1 | Rank1 | nStn | totAssess | totCOMP4 | SpatialRep |
Variable salinity | Diatom | 0 | 4 | 1 | - | 80% | 51% | FALSE | np | 3,5 | 1 | 5 | 9 | 44% |
Dinoflagellate | 1 | 2 | 2 | ↑ | 40% | 51% | FALSE | np | 2,5 | 1 | 5 | 9 | 44% | |
Holoplankton | 1 | 0 | 0 | ↓ | 100% | 30% | FALSE | ph | 1,0 | 0 | 1 | 9 | 11% | |
Meroplankton | 0 | 1 | 0 | - | 100% | 30% | FALSE | psal | 1,0 | 0 | 1 | 9 | 11% | |
Large copepods | 1 | 0 | 0 | ↓ | 100% | 30% | FALSE | sst | 1,0 | 0 | 1 | 9 | 11% | |
Small copepods | 1 | 0 | 0 | ↓ | 100% | 30% | FALSE | sst | 1,0 | 0 | 1 | 9 | 11% | |
Fish larvae | 0 | 0 | 1 | ↑ | 100% | 30% | FALSE | wspd | 1,0 | 0 | 1 | 9 | 11% | |
Gelatinous | 0 | 0 | 0 | NA | NA | 0% | FALSE | NA | NA | 0 | 0 | 9 | 0% | |
Coastal | Diatom | 2 | 9 | 8 | - | 47% | 71% | FALSE | psal | 3,2 | 9 | 19 | 12 | 83% |
Dinoflagellate | 5 | 8 | 6 | - | 42% | 71% | FALSE | ntot | 2,3 | 9 | 19 | 12 | 83% | |
Holoplankton | 3 | 8 | 1 | - | 67% | 59% | FALSE | psal | 2,8 | 2 | 12 | 12 | 83% | |
Meroplankton | 0 | 4 | 8 | ↑ | 67% | 59% | TRUE | sst | 3,1 | 2 | 12 | 12 | 83% | |
Large copepods | 3 | 7 | 2 | - | 58% | 59% | FALSE | precip | 2,1 | 2 | 12 | 12 | 83% | |
Small copepods | 2 | 6 | 4 | - | 50% | 59% | FALSE | sst | 2,2 | 2 | 12 | 12 | 83% | |
Fish larvae | 0 | 6 | 6 | ↑ | 50% | 59% | TRUE | psal | 3,2 | 2 | 12 | 12 | 83% | |
Gelatinous | 0 | 3 | 1 | - | 75% | 64% | FALSE | psal | 1,0 | 2 | 4 | 12 | 17% | |
Shelf | Diatom | 1 | 5 | 5 | ↑ | 45% | 74% | FALSE | phos | 3,2 | 0 | 11 | 11 | 100% |
Dinoflagellate | 7 | 3 | 1 | ↓ | 64% | 74% | TRUE | wspd | 3,9 | 0 | 11 | 11 | 100% | |
Holoplankton | 6 | 4 | 1 | ↓ | 55% | 74% | TRUE | sst | 2,0 | 0 | 11 | 11 | 100% | |
Meroplankton | 0 | 2 | 9 | ↑ | 82% | 74% | TRUE | sst | 2,2 | 0 | 11 | 11 | 100% | |
Large copepods | 3 | 8 | 0 | - | 73% | 74% | FALSE | sst | 2,3 | 0 | 11 | 11 | 100% | |
Small copepods | 4 | 4 | 3 | ↓ | 36% | 74% | FALSE | sst | 1,3 | 0 | 11 | 11 | 100% | |
Fish larvae | 1 | 4 | 6 | ↑ | 55% | 74% | TRUE | attn | 2,7 | 0 | 11 | 11 | 100% | |
Gelatinous | 0 | 0 | 0 | NA | NA | 0% | FALSE | NA | NA | 0 | 0 | 11 | 0% |
In the Celtic Seas (Table k), declining abundance of diatoms in variable salinity habitats have been observed and linked to the increase in the N:P ratio. Declining abundance trends in dinoflagellates, as well as large and small copepods in coastal habitats were linked to temperature increases. Declining abundance of holoplankton in coastal areas may also be linked to decreasing pH. Changes in pH can be linked to changes in primary production, since phytoplankton ingest Dissolved Inorganic Carbon (DIC) to fuel growth and reproduction, but also to the concentration of atmospheric carbon dioxide, the main driver of global warming. In shelf areas a similar increase in meroplankton abundance has been observed in response to temperature, while decreasing abundance of holoplankton and dinoflagellates may be linked to reductions in pH and increases in the N:P ratio, respectively.
Table k: Integration of the indicator results for OSPAR Region III – Celtic Seas. An explanation of column names can be found in the caption for Table j.
Habitat | Lifeform | ↓ | - | ↑ | Dir | Trend | Conf | Change | Press1 | Rank1 | nStn | totAssess | totCOMP4 | SpatialRep |
Variable salinity | Diatom | 2 | 0 | 1 | ↓ | 67% | 70% | TRUE | np | 1,0 | 2 | 3 | 2 | 50% |
Dinoflagellate | 0 | 0 | 3 | ↑ | 100% | 70% | TRUE | attn | 2,5 | 2 | 3 | 2 | 50% | |
Holoplankton | 1 | 0 | 0 | ↓ | 100% | 99% | TRUE | psal | 1,0 | 1 | 1 | 2 | 0% | |
Meroplankton | 1 | 0 | 0 | ↓ | 100% | 99% | TRUE | wspd | 1,0 | 1 | 1 | 2 | 0% | |
Large copepods | 1 | 0 | 0 | ↓ | 100% | 99% | TRUE | sst | 1,0 | 1 | 1 | 2 | 0% | |
Small copepods | 1 | 0 | 0 | ↓ | 100% | 99% | TRUE | amo | 1,0 | 1 | 1 | 2 | 0% | |
Fish larvae | 1 | 0 | 0 | ↓ | 100% | 99% | TRUE | attn | 1,0 | 1 | 1 | 2 | 0% | |
Gelatinous | 1 | 0 | 0 | ↓ | 100% | 99% | TRUE | wspd | 1,0 | 1 | 1 | 2 | 0% | |
Coastal | Diatom | 1 | 2 | 0 | - | 67% | 63% | FALSE | precip | 2,0 | 0 | 3 | 3 | 100% |
Dinoflagellate | 2 | 1 | 0 | ↓ | 67% | 63% | TRUE | sst | 2,0 | 0 | 3 | 3 | 100% | |
Holoplankton | 2 | 1 | 0 | ↓ | 67% | 63% | TRUE | ph | 1,5 | 0 | 3 | 3 | 100% | |
Meroplankton | 0 | 2 | 1 | - | 67% | 63% | FALSE | mld | 2,5 | 0 | 3 | 3 | 100% | |
Large copepods | 2 | 1 | 0 | ↓ | 67% | 63% | TRUE | sst | 1,5 | 0 | 3 | 3 | 100% | |
Small copepods | 2 | 1 | 0 | ↓ | 67% | 63% | TRUE | sst | 1,0 | 0 | 3 | 3 | 100% | |
Fish larvae | 0 | 2 | 1 | - | 67% | 63% | FALSE | mld | 2,5 | 0 | 3 | 3 | 100% | |
Gelatinous | 0 | 0 | 0 | NA | NA | 0% | FALSE | NA | NA | 0 | 0 | 3 | 0% | |
Shelf | Diatom | 1 | 2 | 1 | - | 50% | 87% | FALSE | precip | 2,5 | 0 | 4 | 4 | 100% |
Dinoflagellate | 3 | 1 | 0 | ↓ | 75% | 87% | TRUE | np | 3,0 | 0 | 4 | 4 | 100% | |
Holoplankton | 3 | 1 | 0 | ↓ | 75% | 87% | TRUE | ph | 2,7 | 0 | 4 | 4 | 100% | |
Meroplankton | 0 | 1 | 3 | ↑ | 75% | 87% | TRUE | sst | 1,0 | 0 | 4 | 4 | 100% | |
Large copepods | 1 | 3 | 0 | - | 75% | 87% | FALSE | sst | 1,7 | 0 | 4 | 4 | 100% | |
Small copepods | 1 | 3 | 0 | - | 75% | 87% | FALSE | precip | 1,3 | 0 | 4 | 4 | 100% | |
Fish larvae | 1 | 3 | 0 | - | 75% | 87% | FALSE | psal | 2,7 | 0 | 4 | 4 | 100% | |
Gelatinous | 0 | 0 | 0 | NA | NA | 0% | FALSE | NA | NA | 0 | 0 | 4 | 0% |
In the Bay of Biscay and Iberian Coast region (Table l), holoplankton, large and small copepods in shelf areas are also exhibiting decreasing abundance trends linked to temperature increase, while large and small copepods in oceanic / beyond shelf habitats are experiencing declines linked to increasing temperatures. Trends for variable salinity habitats were not assessed due to the limited data available for this region.
Table l: Integration of the indicator results for OSPAR Region IV – Bay of Biscay and Iberian Coast. An explanation of column names can be found in the caption for Table j.
Habitat | Lifeform | ↓ | - | ↑ | Dir | Trend | Conf | Change | Press1 | Rank1 | nStn | totAssess | totCOMP4 | SpatialRep |
Variable salinity | Diatom | 0 | 0 | 0 | NA | NA | 0% | FALSE | NA | NA | 0 | 0 | 3 | 0% |
Dinoflagellate | 0 | 0 | 0 | NA | NA | 0% | FALSE | NA | NA | 0 | 0 | 3 | 0% | |
Holoplankton | 0 | 0 | 0 | NA | NA | 0% | FALSE | NA | NA | 0 | 0 | 3 | 0% | |
Meroplankton | 0 | 0 | 0 | NA | NA | 0% | FALSE | NA | NA | 0 | 0 | 3 | 0% | |
Large copepods | 0 | 0 | 0 | NA | NA | 0% | FALSE | NA | NA | 0 | 0 | 3 | 0% | |
Small copepods | 0 | 0 | 0 | NA | NA | 0% | FALSE | NA | NA | 0 | 0 | 3 | 0% | |
Fish larvae | 0 | 0 | 0 | NA | NA | 0% | FALSE | NA | NA | 0 | 0 | 3 | 0% | |
Gelatinous | 0 | 0 | 0 | NA | NA | 0% | FALSE | NA | NA | 0 | 0 | 3 | 0% | |
Coastal | Diatom | 0 | 2 | 1 | - | 67% | 52% | FALSE | mld | 1,0 | 0 | 3 | 12 | 25% |
Dinoflagellate | 0 | 0 | 3 | ↑ | 100% | 52% | TRUE | amo | 2,3 | 0 | 3 | 12 | 25% | |
Holoplankton | 0 | 0 | 0 | NA | NA | 0% | FALSE | NA | NA | 0 | 0 | 12 | 0% | |
Meroplankton | 0 | 0 | 0 | NA | NA | 0% | FALSE | NA | NA | 0 | 0 | 12 | 0% | |
Large copepods | 0 | 0 | 0 | NA | NA | 0% | FALSE | NA | NA | 0 | 0 | 12 | 0% | |
Small copepods | 0 | 0 | 0 | NA | NA | 0% | FALSE | NA | NA | 0 | 0 | 12 | 0% | |
Fish larvae | 0 | 0 | 0 | NA | NA | 0% | FALSE | NA | NA | 0 | 0 | 12 | 0% | |
Gelatinous | 0 | 0 | 0 | NA | NA | 0% | FALSE | NA | NA | 0 | 0 | 12 | 0% | |
Shelf | Diatom | 2 | 1 | 0 | ↓ | 67% | 63% | TRUE | wspd | 1,0 | 0 | 3 | 6 | 50% |
Dinoflagellate | 1 | 2 | 0 | - | 67% | 63% | FALSE | np | 2,0 | 0 | 3 | 6 | 50% | |
Holoplankton | 3 | 0 | 0 | ↓ | 100% | 63% | TRUE | sst | 2,3 | 0 | 3 | 6 | 50% | |
Meroplankton | 1 | 1 | 1 | ↓ | 33% | 63% | FALSE | sst | 1,0 | 0 | 3 | 6 | 50% | |
Large copepods | 2 | 1 | 0 | ↓ | 67% | 63% | TRUE | sst | 1,0 | 0 | 3 | 6 | 50% | |
Small copepods | 2 | 1 | 0 | ↓ | 67% | 63% | TRUE | sst | 1,0 | 0 | 3 | 6 | 50% | |
Fish larvae | 0 | 3 | 0 | - | 100% | 63% | FALSE | wspd | 2,0 | 0 | 3 | 6 | 50% | |
Gelatinous | 0 | 0 | 0 | NA | NA | 0% | FALSE | NA | NA | 0 | 0 | 6 | 0% | |
Oceanic / beyond shelf | Diatom | 2 | 0 | 0 | ↓ | 100% | 84% | TRUE | psal | 1,0 | 0 | 2 | 6 | 33% |
Dinoflagellate | 1 | 1 | 0 | ↓ | 50% | 84% | TRUE | psal | 1,0 | 0 | 2 | 6 | 33% | |
Holoplankton | 2 | 0 | 0 | ↓ | 100% | 84% | TRUE | mld | 1,5 | 0 | 2 | 6 | 33% | |
Meroplankton | 0 | 1 | 1 | ↑ | 50% | 84% | TRUE | mld | 1,0 | 0 | 2 | 6 | 33% | |
Large copepods | 2 | 0 | 0 | ↓ | 100% | 84% | TRUE | sst | 1,5 | 0 | 2 | 6 | 33% | |
Small copepods | 2 | 0 | 0 | ↓ | 100% | 84% | TRUE | sst | 1,0 | 0 | 2 | 6 | 33% | |
Fish larvae | 2 | 0 | 0 | ↓ | 100% | 84% | TRUE | mld | 1,5 | 0 | 2 | 6 | 33% | |
Gelatinous | 0 | 0 | 0 | NA | NA | 0% | FALSE | NA | NA | 0 | 0 | 6 | 0% |
Conclusion
Les habitats pélagiques ont considérablement changé au cours des 60 dernières années, avec un déclin généralisé des formes de vie clés dans la majeure partie de l'Atlantique du Nord-Est. Au cours de la courte période d'évaluation elle-même (2015 à 2019), seuls quelques changements ont pu être détectés - il est essentiel de disposer de longues séries chronologiques pour distinguer le changement de la variabilité de fond. La diminution de l'abondance d'importantes formes de vie planctoniques est préoccupante pour l'avenir des réseaux trophiques pélagiques "traditionnels", dans lesquels les efflorescences de phytoplancton nourrissent les copépodes, qui soutiennent les poissons exploités commercialement. À l'inverse, l'augmentation de l'abondance des larves/œufs de poisson et du méroplancton dans toute la mer du Nord pourrait profiter aux pêcheries, mais uniquement si le recrutement augmente avec l'abondance des larves et si les taxons en augmentation sont exploitables.
Les pressions environnementales les plus étroitement liées à l'abondance des formes de vie sont induites par le changement climatique, soit directement (par exemple, changement de la température de l'eau), soit indirectement (par exemple, changement de la profondeur de la couche mélangée). Alors que les changements dans l'abondance des formes de vie dus à d'autres influences anthropogènes (par exemple, l'apport fluvial de nutriments) étaient moins clairs, les résultats suggèrent que d'importants changements dans les habitats pélagiques se sont produits dans les zones côtières, les zones de plateau et les zones océaniques. Compte tenu de l'échelle à laquelle ces pressions s'exercent, il est probable que les mesures de gestion ciblées au niveau régional n'auront dans un premier temps qu'un impact notable sur les eaux côtières et n'auront globalement qu'une incidence minime sur le ralentissement des changements à long terme. Toutefois, des mesures plus généralisées pourraient renforcer la résilience des écosystèmes, même si les effets ne sont pas directement mesurables à de plus grandes distances des côtes, et contribuer ainsi indirectement à ralentir les changements à long terme dans l'avenir.
This assessment indicates spatial and temporal variability in the plankton community for eight ecologically important planktonic lifeforms. While there were clear increasing and decreasing trends in the abundance of lifeforms across the North-East Atlantic, the overall trend was predominantly decreasing for diatoms, dinoflagellates, holoplankton, large copepods and small copepods, particularly within the Celtic Seas and the Bay of Biscay and Iberian Coast (OSPAR Regions III and IV). Meroplankton were the only clear exception, with evidence of increasing abundance across most of the areas assessed, and particularly the Greater North Sea (OSPAR Region II). Evidence of links between trends in lifeform abundance and anthropogenic climate change mainly occurred in shelf and oceanic pelagic habitats, while changes attributed to variation in nutrients were primarily limited to coastal habitats.
This indicator assessment has been conducted in accordance with expert knowledge on plankton dynamics from the scientific literature (McQuatters-Gollop et al., 2019; McQuatters-Gollop et al. 2015). The plankton lifeform indicator has been published in the peer-reviewed literature (McQuatters-Gollop et al., 2019; Tett et al., 2008) and has been developed at the sub-regional scale using data from the pan-European Continuous Plankton Recorder survey which has documented quality assurance procedures and has supported over 1000 scientific publications (McQuatters-Gollop et al., 2015). The methods used here have attempted to improve this established approach, making it more suitable for detecting change linked to environmental pressures.
Links between environmental pressures and plankton lifeforms described here present important areas for further research. There is no single environmental variable linked to change in lifeform abundance, and many of the long-term changes detected in this assessment were unable to be explained by the variables tested. This may be at least partially due to the paucity of robust pressure data available at the spatial and temporal scale and resolution required to conduct a regional assessment of this nature. Local changes may be occurring but are not detectable when considered at a regional scale. It is important to establish clearer causal links by exploring this problem at finer scale than was suitable for a regional assessment, incorporating seasonality and taking better advantage of the high-resolution gridded satellite and other data products currently available, such as abundance of small (nano and pico) plankton which are an important part of the pelagic food web.
As the blue economy continues to develop and industries such as aquaculture are likely to become more important in the coming years, changing plankton communities can impact their sustainability as pelagic habitats change. Further interpretation of results and refinement to the methodology will be essential for understanding the effects these changes can have on ecosystem services, however this will of course be resource dependent.
Lacunes dans les connaissances
Il sera important d’obtenir des séries de données sur le zooplancton dans les habitats pélagiques qui ne sont pas suffisamment couverts par l'évaluation actuelle. D'autres recherches scientifiques seront nécessaires pour explorer les liens directs entre le changement des variables environnementales et le changement de l'abondance des formes de vie. Il pourrait également être utile d'examiner le taux et la direction de l'évolution de l'indice planctonique en ce qui concerne chaque paire de formes de vie, ainsi que les conséquences écologiques de l'évolution de l'abondance des formes de vie sur les niveaux trophiques supérieurs, qui ne sont pas pris en compte dans l'évaluation des habitats pélagiques. Cela pourrait permettre de relier les évaluations des habitats pélagiques et des réseaux trophiques en termes de changements et d'impacts identifiés.
The following work needs to be taken forward to address the knowledge gaps and improve the assessment provided by this indicator:
- Examining national datasets at a finer spatiotemporal scale, which will help establish clearer links between climatic and anthropogenic pressures with changes in pelagic habitats and plankton diversity;
- Introducing lag into the variable selection process to test for delayed effects of environmental pressures on lifeform abundance (e.g., the effects of winter nutrient concentrations on lifeform abundance during the growing season);
- Investigating the impacts of long-term changes in lifeform abundance on pelagic food webs;
- Obtaining pressure datasets that would present an indicator of predation on plankton from higher trophic levels (e.g., forage fish density);
- Obtaining high quality plankton monitoring data for several pelagic habitat types which were underrepresented in this assessment. In particular there is a need for: zooplankton data for variable salinity habitats in the Greater North Sea and Celtic Seas (OSPAR Regions II and III), zooplankton data for coastal habitats and both phytoplankton and zooplankton data for variable salinity habitats in the Bay of Biscay and Iberian Coast (OSPAR Region IV);
- Determination of the traits for many plankton species – for some species even information about basic biological characteristics, such as diet, is not yet known;
- Understanding the role of pico and nanoplankton (small plankton composed by cells with sizes between respectively 0,2 μm and 2 μm, and 2 μm and 20 µm) in the ecosystem. These small size categories are difficult to measure routinely and are thus mainly ignored. However, they make up a significant proportion of the plankton biomass, diversity and trophic roles (De Vargas et al., 2015) relevant to marine food webs and carbon export (Leblanc et al., 2018). Their ecosystem role needs further investigation so that they can be included in an appropriate new or existing lifeform;
- Establishment of monthly monitoring programmes for pico and nanoplankton to provide data on a relatively data-sparse component of the plankton community;
Developing a better understanding for the extent of pico and nanoplankton biological interactions in the context of their environments.
Barnes, M. K., G. H. Tilstone, T. J. Smyth, C. E. Widdicombe, J. Gloël, C. Robinson, J. Kaiser, and D. J. Suggett. 2015. Drivers and effects of Karenia mikimotoi blooms in the western English Channel. Progress in Oceanography 137: 456-469.
Beaugrand, G. 2005. Monitoring pelagic ecosystems using plankton indicators. ICES Journal of Marine Science 62: 333-338.
Bedford, J., C. Ostle, D. G. Johns, A. Atkinson, M. Best, E. Bresnan, M. Machairopoulou, C. A. Graves, M. Devlin, and A. Milligan. 2020a. Lifeform indicators reveal large‐scale shifts in plankton across the North‐West European shelf. Global Change Biology 26: 3482-3497.
Bedford, J., C. Ostle, D. G. Johns, A. Budria, and A. McQuatters-Gollop. 2020b. The influence of temporal scale selection on pelagic habitat biodiversity indicators. Ecological Indicators 114: 106311.
Bremner, J., C. L. J. Frid, and S. I. Rogers. 2004. Biological traits of the North Sea benthos – Does fishing affect benthic ecosystem function? , p. 477-489. In P. Barnes, Thomas, J. [ed.], Benthic habitats and the effects of fishing. American Fisheries Society.
Charvet, S., M. C. Roger, B. Faessel, and M. Lafont. 1998. Biomonitoring of freshwater ecosystems by the use of biological traits. Annals of Limnology – International Journal of Limnology 34: 455-464.
de Jonge, V. N. 2007. Toward the application of ecological concepts in EU coastal water management. Marine Pollution Bulletin 55: 407-414.
De Vargas, C., S. Audic, N. Henry, J. Decelle, F. Mahé, R. Logares, E. Lara, C. Berney, N. Le Bescot, and I. Probert. 2015. Eukaryotic plankton diversity in the sunlit ocean. Science 348: 1261605.
Desmit, X., A. Nohe, A. V. Borges, T. Prins, K. De Cauwer, R. Lagring, D. Van der Zande, and K. Sabbe. 2020. Changes in chlorophyll concentration and phenology in the North Sea in relation to de‐eutrophication and sea surface warming. Limnology and Oceanography 65: 828-847.
Enserink, L., A. Blauw, D. van der Zande, and S. Markager. 2019. Summary report of the EU project 'Joint monitoring programme of the eutrophication of the North Sea with satellite data' (Ref: DG ENV/MSFD Second Cycle/2016), p. 21 pp.
Estrada, M., P. Henriksen, J. M. Gasol, E. O. Casamayor, and C. Pedrós-Alió. 2004. Diversity of Planktonic Photoautotrophic Microorganisms Along a Salinity Gradient as Depicted by Microscopy, Flow Cytometry, Pigment Analysis and DNA-based Methods. FEMS Microbiology Ecology 49: 281-293.
Flynn, K. J., A. Mitra, K. Anestis, A. A. Anschütz, A. Calbet, G. D. Ferreira, N. Gypens, P. J. Hansen, U. John, and J. L. Martin. 2019. Mixotrophic protists and a new paradigm for marine ecology: where does plankton research go now? Journal of Plankton Research 41: 375-391.
Gallego, I., T. A. Davidson, E. Jeppesen, C. Perez-Martinez, P. Sanchez-Castillo, M. Juan, F. Fuentes-Rodriguez, D. Leon, P. Penalver, J. Toja, and J. J. Casas. 2012. Taxonomic or ecological approaches? Searching for phytoplankton surrogates in the determination of richness and assemblage composition in ponds. Ecological Indicators 18: 575-585.
Garmendia, M., Á. Borja, J. Franco, and M. Revilla. 2012. Phytoplankton composition indicators for the assessment of eutrophication in marine waters: Present state and challenges within the European directives. Marine Pollution Bulletin 66: 7-16.
Gowen, R. J., A. McQuatters-Gollop, P. Tett, M. Best, E. Bresnan, C. Castellani, K. Cook, R. Forster, C. Scherer, and A. Mckinney. 2011. The Development of UK Pelagic (Plankton) Indicators and Targets for the MSFD. Advice to Defra, p. 41 pp.
HELCOM. 2012. Development of the HELCOM core-set indicators Part B, GES 8/2012/7b. HELCOM.
Keys, M., G. Tilstone, H. S. Findlay, C. E. Widdicombe, and T. Lawson. 2018. Effects of elevated CO 2 and temperature on phytoplankton community biomass, species composition and photosynthesis during an experimentally induced autumn bloom in the western English Channel. Biogeosciences 15: 3203-3222.
Kursa, M. B., and W. R. Rudnicki. 2010. Feature selection with the Boruta package. Journal of statistical software 36: 1-13.
Leblanc, K., B. Queguiner, F. Diaz, V. Cornet, M. Michel-Rodriguez, X. Durrieu de Madron, C. Bowler, S. Malviya, M. Thyssen, and G. Grégori. 2018. Nanoplanktonic diatoms are globally overlooked but play a role in spring blooms and carbon export. Nature Communications 9: 1-12.
Mayer, M., and M. M. Mayer. 2019. Package ‘missRanger’. R Package.
McQuatters-Gollop, A., A. Atkinson, A. Aubert, J. Bedford, M. Best, E. Bresnan, K. Cook, M. Devlin, R. Gowen, and D. G. Johns. 2019. Plankton lifeforms as a biodiversity indicator for regional-scale assessment of pelagic habitats for policy. Ecological Indicators 101: 913-925.
McQuatters-Gollop, A., M. Edwards, P. Helaouët, D. G. Johns, N. J. Owens, D. E. Raitsos, D. Schroeder, J. Skinner, and R. F. Stern. 2015. The Continuous Plankton Recorder survey: How can long-term phytoplankton datasets contribute to the assessment of Good Environmental Status? Estuarine, Coastal and Shelf Science 162: 88-97.
McQuatters-Gollop, A., L. Guérin, N. L. Arroyo, A. Aubert, L. F. Artigas, J. Bedford, E. Corcoran, V. Dierschke, S. A. M. Elliott, S. C. V. Geelhoed, A. Gilles, J. M. González-Irusta, J. Haelters, M. Johansen, F, C. P. Lynam, N. Niquil, B. Meakins, I. Mitchell, B. Padegimas, R. Pesch, I. Preciado, I. Rombouts, G. Safi, P. Schmitt, U. Schückel, A. Serrano, P. Stebbing, A, and C. Vina-Herbon. 2022. Assessing the state of marine biodiversity in the Northeast Atlantic. Ecological Indicators 141: 109148.
Mouillot, D., S. Spatharis, S. Reizopoulou, T. Laugier, L. Sabetta, A. Basset, and T. D. Chi. 2006. Alternatives to taxonomic-based approaches to assess changes in transitional water communities. Aquatic Conservation-Marine and Freshwater Ecosystems 16: 469-482.
Ostle, C., K. Paxman, C. A. Graves, M. Arnold, L. F. Artigas, A. Atkinson, A. Aubert, M. Baptie, B. Bear, and J. Bedford. 2021. The Plankton Lifeform Extraction Tool: a digital tool to increase the discoverability and usability of plankton time-series data. Earth System Science Data 13: 5617-5642.
Rakocinski, C. F. 2012. Evaluating macrobenthic process indicators in relation to organic enrichment and hypoxia. Ecological Indicators 13: 1-12.
Reid, P. C., J. Colebrook, J. Matthews, J. Aiken, and C. P. R. Team. 2003. The Continuous Plankton Recorder: concepts and history, from Plankton Indicator to undulating recorders. Progress in Oceanography 58: 117-173.
Svendsen, L., L. van der Bijl, S. Boutrup, and B. Norup. 2005. NOVANA. National Monitoring and Assessment Programme for the Aquatic and Terrestrial. and number: NERI Technical Report.
Tett, P., C. Carreira, D. K. Mills, S. van Leeuwen, J. Foden, E. Bresnan, and R. J. Gowen. 2008. Use of a phytoplankton community index to assess the health of coastal waters. ICES Journal of Marine Science 65: 1475-1482.
Contributeurs
Auteurs principaux : Matthew Holland, Arnaud Louchart, Luis Felipe Artigas and Abigail McQuatters-Gollop.
Avec le soutien des groupes suivants : UK Pelagic Habitats Expert Group, Intersessional Correspondence Group on the Coordination of Biodiversity Assessment and Monitoring, OSPAR Pelagic Habitats Expert Group, Intersessional Correspondence Group on Eutrophication, Intersessional Correspondence Group on the Quality Status Report, Biodiversity Committee and the OSPAR Secretariat.
Réalisation de l’évaluation
Ce travail a été co-financé par le Fonds européen pour les affaires maritimes et la pêche dans le cadre du projet : « North-East Atlantic project on biodiversity and eutrophication assessment integration and creation of effective measures (NEA PANACEA) », financé par la DG ENV/MSFD 2020 de l'Union européenne, dans le cadre de l'accord n° 110661/2020/839628/SUB/ENV.C.2.
![]() | ![]() |
Citation
Holland, M., Louchart, A., Artigas, L. F. and McQuatters-Gollop, A. 2023. Modifications des communautés phytoplanctoniques et zooplanctoniques. OSPAR, 2023 : Bilan de santé de l’Atlantique du Nord-Est. Commission OSPAR, Londres. Disponible via le lien suivant : https://oap.ospar.org/fr/ospar-assessments/quality-status-reports/qsr-2023/evaluations-des-indicateurs/modifications-phytoplanctoniques-zooplanctoniques/
Type d’évaluation | Évaluation d’indicateur |
---|---|
Résumé des résultats | https://odims.ospar.org/en/submissions/ospar_changes_phy_zoo_msfd_2022_06_001/ |
Indicateur ODD | 14.2 By 2020, sustainably manage and protect marine and coastal ecosystems to avoid significant adverse impacts, including by strengthening their resilience, and take action for their restoration in order to achieve healthy and productive oceans |
Activité thématique | Diversité biologique et écosystèmes |
Documentation OSPAR pertinente | OSPAR Agreement 2010-03 The North-East Atlantic Environment Strategy. Strategy of the OSPAR Commission for the Protection of the Marine Environment of the North-East Atlantic 2010–2020 |
Date de publication | 2023-06-30 |
Conditions d’accès et d’utilisation | https://oap.ospar.org/en/data-policy/ |
Instantané de données | https://odims.ospar.org/en/submissions/ospar_changes_phy_zoo_dsnap_2022_06_001/ |
Instantané de données | https://odims.ospar.org/en/submissions/ospar_changes_phy_zoo_dsnap_2022_07_001/ |
Résultats des données | https://odims.ospar.org/en/submissions/ospar_changes_phy_zoo_dres_2022_06_001/ |
Source des données | https://www.dassh.ac.uk/lifeforms/ |